Research-Based Teaching
Integrating research infrastructures into teaching
Our needs analysis revealed that linguistics and language-related degree programmes seldom include language data standards and research data repositories in their learning outcomes. A survey of lecturers from linguistics and language-related disciplines also exposed a number of challenges in using repositories for language data discovery, reuse and archiving. Against this backdrop, the present guide shows how teachers and trainers can leverage the CLARIN research infrastructure to help students enhance their data collection, processing and analysis, and archiving skills. By integrating research infrastructures into teaching, educators can bridge the gap between theoretical knowledge and practical aspects of linguistic research data management, equipping students with the necessary skills and competences to thrive in the evolving landscape of open science and data-driven research.
The motivation for writing this guide arises from several factors identified within UPSKILLS’s remit. The lecturers who participated in our needs analysis expressed interest in integrating research infrastructure data and services into their teaching. However, they faced some challenges, such as difficulty in identifying optimal resources and tools to explore specific linguistic aspects, lack of resources for certain languages, insufficient documentation and tutorials on how to effectively incorporate resources in the classroom, and the absence of discipline-specific best practices and guidelines. An additional questionnaire conducted as part of Intellectual Output 2 revealed some technical, financial, and administrative challenges that both lecturers and students encountered when using repositories for language data discovery, reuse and archiving. Furthermore, students’ low level of digital literacy was cited as a barrier to using infrastructure and tools in data collection and archiving.
At the same time, informal talks with the UPSKILLS consortium partners and lecturers outside the consortium revealed a lack of general awareness about the wealth of language resources and knowledge available through the CLARIN research infrastructure and national consortia in their respective countries. This lack of general awareness about the existence and added value of research infrastructures has also been acknowledged at the European level by initiatives such as EOSC and FAIRsFAIR. A university landscape analysis conducted by the EOSC Skills and Training Working Group in 2019 identified a low awareness among students and researchers of research data management (RDM) practices, a lack of skills and insufficient training opportunities at the bachelor, master and doctoral levels (Stoy et al., 2020). Although things have improved gradually at the PhD level, there is evidence of slow integration of FAIR data principles, open science and data-related topics at the Bachelor and Master levels. Therefore, the FAIR Competence Framework for Higher Education proposes a set of core competences for FAIR data education that universities can use to design and integrate RDM and FAIR-data-related skills in their curricula and programmes (Demchenko et al., 2021). Students, scholars, teachers and researchers from all disciplines are encouraged to acquire fundamental skills for open science, including the ability to effectively interact with federated research infrastructures and open science tools for collaborative research. To further support the integration of these skills into the university curricula, FAIRsFAIR published an adoption handbook “How to be FAIR with your data – A teaching and training handbook for higher education institutions’’ (Engelhardt et al., 2022), which contains ready-made lessons plans on a variety of topics, including the use of repositories, data creation and reuse. We hope this CLARIN guide and learning content is a useful addition to these European initiatives but from a discipline-specific angle.
As Gledic et al. (2021) reveal, employers in the digital business sector increasingly seek to hire graduates from language-related programmes with data-oriented and research-oriented skills. Such skills have become even more important as new job profiles continue open up to language and linguistic graduates in the age of AI and ChatGPT revolution, such as computational linguists, machine translation specialists, data curators, data stewards, data annotators, knowledge engineers and terminologists.
In light of this current context, this guide shows how teachers and trainers can leverage the CLARIN infrastructure to help students enhance their data collection, processing and analysis, and archiving skills. By integrating research infrastructures into teaching, educators can bridge the gap between theoretical knowledge and practical aspects of linguistic research data management, equipping students with the necessary skills and competences to thrive in the evolving landscape of open science and data-driven research.
👉 PRO-TIP: See the interactive website that the consortium partners from the University of Bologna developed to help students identify the skills they need for the job profiles they targeted. |
References:
- Demchenko, Yuri, Lennart Stoy, Claudia Engelhardt, and Vinciane Gaillard. ‘D7.3 FAIR Competence Framework for Higher Education (Data Stewardship Professional Competence Framework)’, 24 February 2021. DOI: 10.5281/zenodo.5361917.
- Engelhardt, C., K. Biernacka, A. Coffey, R. Cornet, A. Danciu … B. Zhou (2022). How to be FAIR with your data. A teaching and training handbook for higher education institutions (V1.2.1) [Computer software]. Zenodo. DOI: 10.5281/zenodo.6674301
- Gledić, J., M. Đukanović, M. Miličević Petrović, I. van der Lek & S. Assimakopoulos. (2021). Survey of curricula: Linguistics and language-related degrees in Europe. Zenodo. DOI: 10.5281/zenodo.5030861
- Stoy, L., B. Saenen, J. Davidson, C. Engelhardt & V. Gaillard. (2020). FAIR in European Higher Education (1.0). Zenodo. DOI: 10.5281/zenodo.5361815
This guide complements the Research-Based Teaching: Guidelines and Best Practices by providing a practical introduction to the CLARIN research infrastructure for lecturers, teachers, trainers and curriculum designers interested in integrating learning resources (e.g. corpora), language technology tools and research data repositories in their curricula, summer schools, and workshops. Along with this guide, an accompanying learning block titled Introduction to Language Data: Standards and Repositories is available on Moodle as part of Task 3.2. UPSKILLS Learning Content. This learning block consists of a modular structure that provides lecturers with a comprehensive collection of learning resources and activities they can use to educate themselves and as reference materials for the classroom. Each major topic in this guide links to relevant learning resources on Moodle to help the teachers identify what they need.
Teachers and trainers can use the guide in the following ways:
- To identify CLARIN centres of expertise, research data repositories, language resources (mainly corpora), services and natural language processing (NLP) tools that they can use to enhance their research and teaching.
- To identify learning content and activities in the accompanying course on Moodle, which they can use in two ways: (1) further educate themselves on a specific topic and (2) repurpose for classroom use to help students improve their data discovery, handling, sharing and archiving skills.
👉 PRO-TIPS:
|
Teachers and trainers who already use the CLARIN infrastructure for language research or research data management in their courses are encouraged to share and contribute to future versions of this guide with their teaching and learning activities examples.
While aimed primarily at teachers and trainers in linguistics and language-related fields, this guide can also benefit anyone in humanities and social sciences disciplines, including curriculum designers, policymakers, librarians, data stewards, and industry professionals seeking to use the infrastructure for research and training.
This work in UPSKILLS aligns with international initiatives like the European Open Science Cloud (EOSC) and FAIRisFAIR, which promote the adoption of open science and research data management based on the FAIR guiding principles (Wilkinson et al., 2016) for scientific data management across all domains, disciplines and levels.
Please note this guide does not aim to teach how to design, plan and evaluate a course. To this effect, teachers, instructors and curriculum designers may benefit from other guidelines developed in the project.
👉 PRO-TIPS:
|
*A PDF version of this guide is available for download in the Zenodo repository.
** Throughout this guide, you will encounter many technical terms. A glossary of terms can be found in our Moodle learning block, and it can also be downloaded from UPSKILLS Glossary – Introduction to Language Data – Standards and Repositories.
References:
- Gledić, J., A. Assimakopoulos, I. Buchberger, J. Budimirović, M. Đukanović, T. Kraš, M. Podboj, N. Soldatić & M. Vella, Michela. (2021). UPSKILLS guidelines for Learning Content Creation. Zenodo. DOI: 10.5281/zenodo.8302296
- Simonović, M, I. van der Lek, D. Fišer & B. Arsenijević, B. (2021). Guidelines for the students’ projects and research reporting formats. Zenodo. DOI: 10.5281/zenodo.8297430
- Simonović, M., B. Arsenijević, I. van der Lek, S. Assimakopoulos, L. ten Bosch, D. Fišer, T. Kraš, P. Marty, M. Miličević Petrović, S. Milosavljević, M. Tanti, L. van der Plas, M. Pallottino, G. Puskas & T. Samardžić. (2023). Research-based teaching: Guidelines and best practices. Zenodo. DOI: 10.5281/zenodo.8176220
- Wilkinson, M. D., M. Dumontier, I.J. Aalbersberg, G. Appleton, M. Axton, A. Baak, … B. Mons, B. (2016). The FAIR Guiding Principles for scientific data management and stewardship. Scientific Data, 3, 160018–160018. DOI: 10.1038/sdata.2016.18
The European Commission defines research infrastructures as:
Facilities, resources and services used by the science community to conduct research and foster innovation. They include major scientific equipment, resources such as collections, archives or scientific data, e-infrastructures such as data and computing systems, and communication networks. They can be used beyond research, e.g. for education or public services and they may be single-sited, distributed, or virtual (European Commission, 2016).
Research infrastructures (RIs) are based on national consortia of research institutes, universities, libraries, museums and archives and support researchers in managing the “data lifecycle” in their research projects by providing guidelines for the creation of data management plans and formats to facilitate long-term preservation, access and reuse of research data in the context of Open Science, Open Access and FAIR data principles. The availability of open research data offers valuable resources (e.g. digital text collections, corpora) for students and educators to explore and analyse real-world datasets and it helps them engage in collaborative research across various disciplines. The Helsinki Digital Humanities Hackathon represents a great example of interdisciplinary collaboration, data-driven research, and teaching. Many educational institutions and organisations across Europe have recognised the importance of open science and have incorporated training programs and courses to educate students and researchers about open science principles.
👉 PRO-TIP: To help students understand the benefits of open science, encourage them to play the Open Up Your Research Game developed by the University of Zurich. The game scenario centres around a PhD candidate who needs to choose between adopting an open science or a traditional approach to conducting research. |
Since 2002, about 50 European research infrastructures (RIs) have been set up under the auspices of the European Strategy Forum on Research Infrastructures (ESFRI) in a wide variety of disciplines: e-Infrastructures, Energy, Environment, Health and Food, Physical Sciences & Engineering, and Social and Cultural Innovation. Examples of research infrastructures in the Social and Cultural Innovation sector are CESSDA, CLARIN ERIC, DARIAH-EU, E-RHIS, ESS, and SHARE (for more details, see the ESFRI Roadmap). These European RIs aim to provide open, fair and transparent access to their facilities and services for researchers, scholars and students across Europe and beyond. Moreover, in 2018, the European Commission launched the European Open Science Cloud (EOSC) initiative, which aims to aggregate the services provided by these research infrastructures into one open virtual environment that shares scientific data across borders and disciplines.
👉 PRO-TIP: To learn how the CLARIN services are integrated into the EOSC platform, watch this video: A Study on the Use of Nouns by Female and Male Members of Parliament. Teachers can replicate this small study with their MA or PhD students using this tutorial from the CLARIN website. |
The two most relevant research infrastructures in digital humanities are the CLARIN ERIC and DARIAH-EU infrastructures. While DARIAH-EU has a broader focus on the arts and humanities disciplines, facilitating knowledge exchange and collaboration through working groups, CLARIN ERIC focuses on the collection, management and long-term archiving of language resources and technologies in social sciences and humanities. These two infrastructures collaborate closely on various topics related to training and education through the Digital Humanities Course Registry working group, and by supporting and co-organising summer schools and workshops in digital humanities. This guide will mainly focus on CLARIN and how teachers can use it for language and linguistic research.
Besides CLARIN, there are also other infrastructures used to discuss, host and disseminate language resources and technologies, such as ELRC-SHARE, European Language Resources Association (ELRA), European Language Grid (ELG), and META-SHARE. For more details and the state-of-the-art on language resources and technology developments, refer to Agerri et al. (2023).
📖 Teaching & Learning Resources on Moodle To give students a general introduction to research infrastructures for language resources and technologies, you can use and combine the following learning activities on Moodle, Introduction to Language Data: Standards and Repositories:
Note: The numbers of the Moodle activities are not chronological because the content has been combined from several units to create learning paths. |
References:
- Agerri, R., E. Agirre, I. Aldabe, N. Aranberri, J.M. Arriola, A. Atutxa, G. Azkune, J.A. Campos, A. Casillas, … A. Soroa. (2023). State-of-the-Art in language technology and language-centric Artificial Intelligence. In Rehm, G., Way, A. (Eds) European Language Equality: Cognitive Technologies. Cham: Springer.
- European Commission, Directorate-General for Research and Innovation. (2016) European charter of access for research infrastructures: principles and guidelines for access and related services. Publications Office. DOI: https://data.europa.eu/doi/10.2777/524573
To ensure that language resources and datasets can be found, are accessible, interoperable and reusable, researchers are recommended to deposit language resources, tools and associated metadata in a FAIR and trustworthy research data repository. FAIR is a set of guiding principles for scientific data management developed by Wilkinson et al. (2016) to help improve the findability, accessibility, interoperability and reuse of digital assets. These principles have started to be adopted for data, software, and even training materials. FAIR repositories help researchers make their language resources findable and accessible for a long time by assigning persistent identifiers (PID), such as DOI or handle, which will help retrieve a resource even when it has been moved to another server or domain. Furthermore, repositories provide a fixed set of metadata elements (or schema) to describe the deposited language resources consistently, e.g. using Dublin Core, OLAC, or CMDI. A free metadata search engine (e.g. Virtual Language Observatory or OLAC) may be included in the infrastructure to allow users to search and locate language resources useful for a specific (research project). In addition, repositories use standardised authentication and authorisation procedures (e.g. single-sign-on) and support different licence models to enable controlled access permissions for different user groups (i.e. use a resource for academic or commercial purposes). Finally, repositories promote interoperability through open and standardised formats and facilitate reusability of the language resources and datasets by providing guidelines and best practices for research data management. To ensure that a research data repository is trustworthy, it must undergo a quality assessment procedure and get a seal of quality, e.g. CoreTrustSeal, CLARIN B-Centre Certificate, ISO Standard 16363:2012. Data repositories can also serve as backups during rare but devastating events where data is lost to the researcher and must be retrieved.
👉 PRO-TIP: Go to the CLARIN Centre Registry and see if you can find a CoreTrustSeal-certified centre in your country. |
For teachers and students who have never used research data repositories in research or teaching, the re3data repository registry is a good platform to start exploring available linguistic data repositories. This cross-disciplinary directory contains the metadata of over 2000 repositories in different disciplines. Each repository entry is described with a consistent set of metadata and it can be cited. Here is a quick guide on how to use the registry:
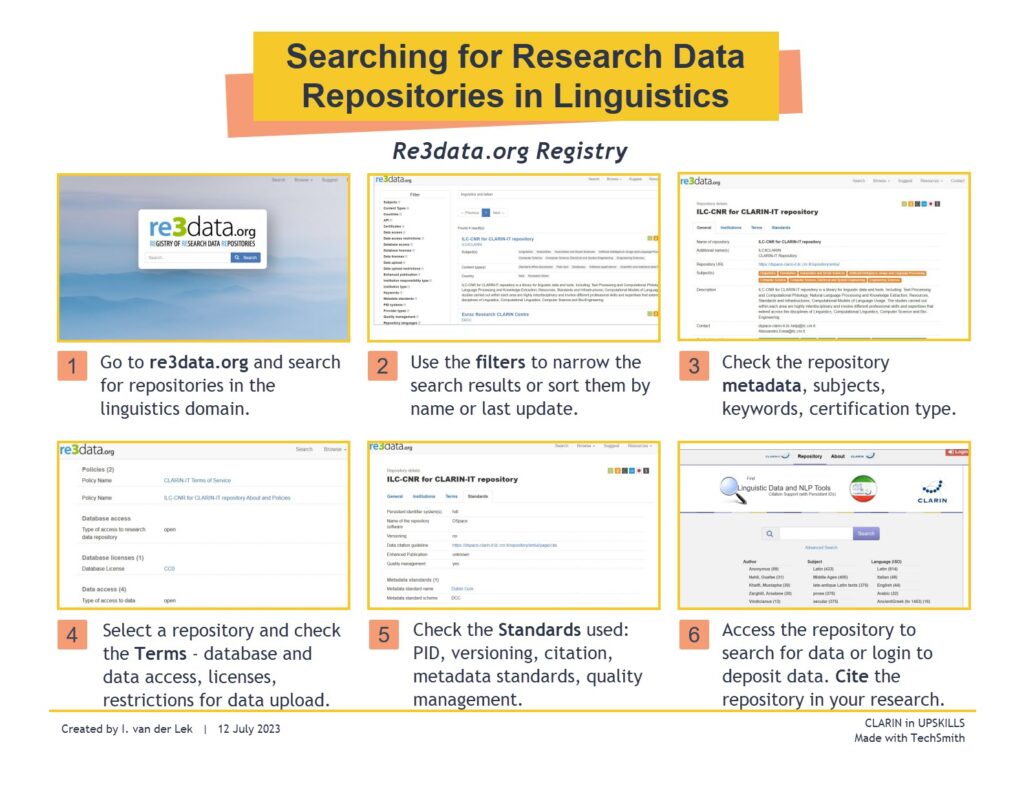
Searching for linguistic data repositories in the re3org. repository registry
When searching for a repository to use for either linguistic research or for sharing and archiving research outputs, teachers could use the re3data registry to teach students how to evaluate and compare different linguistic research data repositories using the checklist that follows:
-
- What types of policies, documentation and guidelines does the repository offer?
- Is the repository certified (e.g. CoreTrust Seal or CLARIN certification)?
- Can users search for and access the research data in open access, or are they required to register?
- Are registration and membership required to be able to upload one’s research data to the repository?
- What type of licences does the repository support? (e.g. Creative Commons Licences)
- Does it support common metadata standards and formats (e.g. Dublin Core)?
- Does it support citation standards and attribution (e.g. implementation of persistent identifiers, such as handles and/or DOIs)?
- Does the repository offer documentation, guidelines and tutorials for finding and using research data?
- Does the repository support the integration of language resources, tools and services in educational settings?
- Does the repository comply with ethical guidelines for data sharing, especially when dealing with sensitive or personal information?
Through the questionnaire in Annex B (please see the PDF version for details) and testimonials of the lecturers participating in the UPSKILLS projects and events, we have collected a few examples of repositories used for teaching purposes in different language-related disciplines. Student teaching assistants who start teaching may find them useful as these repositories provide valuable resources for both teaching and research purposes.
The IRIS database, the ReLDI repository for data collection instruments, or the SLA Speech Tools repository are often used to search and download learning activities to help students improve their pronunciation in the classroom. The CMU-TalkBank repositories, which give access to well-documented CHILDES corpora are used to teach students how to analyse child language corpora. For example, the corpora have been widely used in undergraduate courses in language development to create handouts containing sample transcripts that students need to analyse and answer specific questions about language, or make more thorough analysis at the phonological, morphosyntax and discourse levels. Other teachers use the CHAT transcription program to teach students how to correct transcription errors or collect, record and transcribe child language data themselves. For more examples, please refer to the CHILDES Teaching Resources. For parallel corpora, terminology databases or research tools for the study of translated texts, take a closer look at the CLARIN repositories, EuroParl, ELRA-ELDA catalogues, META-SHARE, EuroTermBank, and TAPoR.For example, these repositories can be used to find and download parallel corpora in .tmx format to use for a translation assignment in the Computer-Aided Translation classroom, extract domain-specific terminology to create a bilingual glossary or use the corpus to train a machine translation engine in AI/Machine Learning programmes. Furthermore, many corpora available in CLARIN are directly integrated into concordancers, such as NoSketch Engine, KonText, Corpuscle, and Korp, which can be freely used in the classroom for linguistic research. To search for speech data and tools, the following repositories may be useful: the Bavarian Archive for Speech Signals (BAS), Speech Data & Technology platform, Open SLR,, and the Linguistic Data Consortium. The BAS repository shares speech resources of contemporary German and detailed information about what standards to use when compiling speech corpora and templates for informed consent forms for speakers participating in a research project. Moreover, the Speech Data and Tech platform offers a Transcription Portal for automatic transcription in English, German, Dutch and Italian. The ELAR (Endangered Language Archives) repository provides multimedia collections of endangered languages from all over the world, which can be browsed free of charge. For more advanced use of the resources, registration is needed. The archive also provides documentation, guidelines and training on research data management, using ELAN to create, transcribe and translate files and Lameta to create consistent quality metadata.blank
First and Second Language Acquisition
Translation Studies and Translation Technology Research
Language and Speech Technologies
Language Documentation
After identifying a suitable repository, teachers are advised to check whether it offers integrated, easy-to-use services and tools that can be used in the classroom and whether the repository has an active user community and provides training and support to its users, e.g.
-
- Does the repository provide training materials, workshops and webinars to teachers and trainers on how to use the services in education and training?
- Does the repository have an active user community? Do other colleagues use it in your field for research or teaching?
- Would the repository provide support if you were to develop a language resource with your students as part of a research project?
Once teachers become familiar with research data repositories and their services, they can help students choose suitable repositories for their projects, data type and research goals.
📖 Teaching and Learning Resources on Moodle To introduce students to research data repositories and the FAIR data principles, the following teaching resources can be used: Introduction to Language Data: Standards and Repositories
|
References:
- Wilkinson, M. D., M. Dumontier, I.J. Aalbersberg, G. Appleton, M. Axton, A. Baak, … B. Mons, B. (2016). The FAIR Guiding Principles for scientific data management and stewardship. Scientific Data, 3, 160018–160018. DOI: 10.1038/sdata.2016.18
After a short overview of the current research infrastructure landscape, this section introduces the CLARIN research infrastructure, an established European Research Infrastructures for “Common Language Resources and Technology Infrastructure.” CLARIN offers online access to an extensive range of written, spoken, or multimodal language resources, which can be used for research, training and education, and developing language technology applications. For an overview of the state-of-the-art in language technology (LT) development, refer to Agerri et al. (2023).
The term linguistic resource refers to (usually large) sets of language data and descriptions in machine-readable form, to be used in building, improving, or evaluating natural language (NL) and speech algorithms or systems. Examples of linguistic resources are written and spoken corpora, lexical databases, grammars, and terminologies, although the term may be extended to include basic software tools for the preparation, collection, management, or use of other resources. (Godfrey & Zampolli, 1997, p. 441)
The infrastructure operates through a distributed network of centres and services, allowing academic users from various fields, particularly in the humanities and social sciences, to use integrated applications to discover, explore, exploit, annotate, analyse or combine language datasets to answer new research questions. To promote accessibility and usability, all CLARIN repositories and services adhere to the Open Science and FAIR data principles (Wilkinson et al., 2016), making the deposited language data findable, accessible, interoperable and reusable.
If have never used the CLARIN infrastructure in research and/or teaching, you may want to watch this short introductory video with your students:
Member countries contribute to the ERIC financially and in kind, for instance, by hosting a CLARIN centre. Researchers, students and teachers from the member countries have access to several central core services and opportunities, which will be showcased throughout this guide. Users from non-member countries (e.g. Serbia, Slovakia, Malta, USA) can also access and explore all the central services and the metadata of the language resources and tools in the repositories freely, without having to log in.
👉 PRO-TIP: Check the list of participating consortia to learn if your country is a member of CLARIN. If your country is not a member of CLARIN, but you are interested in using resources with restricted access or depositing language resources in a CLARIN repository, please contact the central CLARIN office at clarin@clarin.eu. |
References:
- Agerri, R., E. Agirre, I. Aldabe, N. Aranberri, J.M. Arriola, A. Atutxa, G. Azkune, J.A. Campos, A. Casillas, … A. Soroa. (2023). State-of-the-Art in language technology and language-centric Artificial Intelligence. In Rehm, G., Way, A. (Eds) European Language Equality: Cognitive Technologies. Cham: Springer.
- Godfrey, J.J. & A. Zampolli. (1997). Language resources. In A. Zampolli & G. Battista Varile (Eds) Survey of the State of the Art in Human Language Technology. Linguistica Computazionale, XII-XIII, pp. pages 381–384. Pisa: Giardini Editori e Stampatori (also Cambridge University Press)
- Wilkinson, M. D., M. Dumontier, I.J. Aalbersberg, G. Appleton, M. Axton, A. Baak, … B. Mons, B. (2016). The FAIR Guiding Principles for scientific data management and stewardship. Scientific Data, 3, 160018–160018. DOI: 10.1038/sdata.2016.18
The technical infrastructure ensures that academic users in all participating countries can discover and use the language resources made available and hosted by the various local data centres through a single sign-on access using a federated identity (i.e. your university credentials or your CLARIN account).
-
- All users can freely explore the CLARIN core services to search for language resources (data and tools) and expertise on specific language research and documentation topics.
- Due to license restrictions, some resources and services are only available for academic use. To access these resources, login is required through the CLARIN Service Provider Federations, using your institutional or CLARIN website credentials.
- If your university or academic institute is not listed in the list of organisations, you can request a CLARIN account here.
If help is required to access specific corpora, please check the articles on the CLARIN Knowledge Base.
📖 Teaching and Learning Resources on Moodle Introduction to Language Data: Standards and Repositories: To introduce students to the CLARIN research infrastructure, the following learning content from Moodle can be used: Presentations:
Assignment:
|
👉 PRO-TIP: To learn more about CLARIN and its history, we recommend these two articles from the CLARIN anniversary book:
|
This section gives an overview of the national CLARIN Knowledge Centres (K-centres), which the UPSKILLS consortium partners and educators may benefit from. These knowledge centres provide expertise, guidance and training on linguistic topics, data types and tools available via the infrastructure, language processing and linguistic research data management.
Use the keyword-based search or the direct links below to locate those K-centres with expertise in language resources and methods that might be relevant to your teaching and research area:
-
- Individual languages (e.g. Danish, Czech, Portuguese), language families (e.g. South Slavic) or groups of languages (e.g. morphologically rich languages, the languages of Sweden)
- Written text and modalities other than written text (e.g. spoken language, sign language)
- Linguistic topics (e.g. language diversity, language learning, diachronic studies)
- Language processing topics (e.g. speech analysis, building treebanks, machine translation)
- Data types other than corpora (e.g. lexical data, word nets, terminology banks)
- Using or processing families of language data that will exist for most languages (e.g. newspapers, parliamentary records, oral history)
- Generic methods and issues (e.g. data management, ethics, intellectual property rights, digitalisation of texts using optical character recognition technology)
Below, we highlight a few CLARIN K-Centres located in the countries of the UPSKILLS consortium partners to help raise awareness about the expertise of these centres and their added value for research and teaching. A full overview of all the CLARIN centres can be found here.
In Austria, there are two knowledge centres: In Germany, SAW Leipzig, a Text + / CLARIN Centre, focuses on preserving language and lexical resources for underrepresented languages and offers various research-based applications to explore them. For example, the Vocabulary Portal gives access to over 30 million sentences of German-language newspaper corpora crawled from the Web. In addition, the centre offers a corpora portal that offers a search interface to more than 1000 corpus-based monolingual dictionaries in 293 languages. The corpora can be downloaded. The platform can be used to search for words with similar context, examples of use, and neighbour cooccurrences and visualise the relations in a word graph. The CLARIN Knowledge Centre for Computer-Mediated Communication and Social Media corpora provides researchers and students with knowledge, support and training for developing and managing CMC corpora. More specifically, the centre provides FAIR guidelines on data management, such as using standards and formats, and advice on legal and ethical issues. Researchers, teachers and students can contact the centre via their helpdesk. More information about CMC corpora and how to work with this type of corpora is provided in 4.1.3.4. Computer-Mediated Corpora. ILC4CLARIN.IT offers services for browsing and querying corpora, file conversion, term extraction, lexical editing and text annotation, mainly for Italian languages. Teachers of syntax and morphology can use language resources and training materials from INESS, the Norwegian Infrastructure for the Exploration of Syntax and Semantics. This centre is part of the CLARINO Bergen Centre and the CLARIN Knowledge Centre for Treebanking. It provides access to treebanks that are databases of syntactically and semantically annotated sentences. The platform is language-independent and can be used for building, accessing, searching and visualising treebanks. Data from INESS has also been used in a Master’s course on computational language models to show how empirical corpus data can strengthen or challenge hypotheses about grammar. Students quickly learn to use the system in projects for term papers and master’s theses. Victoria Troland’s master’s thesis, for instance, used INESS to extract syntactic markers from syntactically analysed Norwegian novels and subsequently used these markers as a basis for an author identification model. To teach linguists how to query treebanks, see the INESS Search Walkthrough and the Parseme tutorial: Studying MWE annotations in treebanks. The CLASSLA K-centre provides expertise and training on developing language resources and technologies for South Slavic Languages, including Slovenian, Slovene, Croatian, Bosnian, Serbian, Montenegrin, Macedonian, and Bulgarian. The platform gives access to research and tools for language processing, such as information extraction, language understanding, named entity recognition, processing of morphologically rich languages and speech recognition. The centre is managed by CLARIN.SI, the Institute of Croatian Language and Linguistics, and CLADA.BG. Moreover, it offers documentation on how to use the CLARIN.SI infrastructure in Slovene, Croatian and Serbian. A detailed description of the centre is available in Tour de CLARIN. Teachers and researchers of bilingual language development and sign language are referred to the CLARIN K-centre for Atypical Communication Expertise (ACE), hosted by Radboud University in Nijmegen. Internationally, the centre is linked to the DELAD task force, an initiative that provides guidelines for data acquisition, processing and sharing of corpora and datasets that contain sensitive data (e.g. speech, audio and transcripts collected from people with language disorders). Moreover, the centre collaborates closely with both the Language Archive and the TalkBank repositories to host and give access to corpora of speech disorders securely and in a GDPR-compliant way. Educators can find some examples of well-documented speech corpora on the K-centre website, which can be used to teach students how to analyse disordered speech. Conversely, researchers can use the available corpora to refine the analysis methods and formulate and test hypotheses. For teaching and learning materials related to the impact of GDPR on language research and how to handle sensitive research data collected from human subjects, see this impact story: Navigating GDPR with Innovative Educational Materials. Students and researchers working with patient data learn how to perform a Data Protection Impact Assessment (DPIA) through role plays and use cases. 📖 Teaching and Learning Resources on Moodle To make students aware of key ethical issues in data collection and sharing of pathological speech data, see the guidelines on the DELAD website and use the following tutorial on Moodle: Several universities that are part of the CLARIN-CH consortium, develop language resources, datasets, and tools and provide expertise and training in language sciences. Teachers and students, searching for language resources, are referred to the collection of CLARIN-CH Resources and the national Linguistic Corpus Platform hosted by LiRI. Here, we highlight the computer-mediated communication (CMC) corpora collected in the What’s up, Switzerland? project. It contains 617 WhatsApp chats in all four national languages of Switzerland and their varieties, and they are freely available for linguistic research. To learn how to use and query the corpus, see the project website: https://whatsup.linguistik.uzh.ch/start. This project is also a good example for students and researchers who want to learn how to process, handle and annotate CMC corpora. blank
Austria
Germany
Italy
Norway
👉 PRO-TIP: To learn how to query linguistically annotated corpora, see Kuebler, S., & H. Zinsmeister. (2014). Corpus Linguistics and Linguistically Annotated Corpora, London: Bloomsbury. Retrieved from https://ebookcentral.proquest.com/lib/uunl/detail.action?docID=1840024
South-Slavic Countries
👉 PRO-TIP: In June 2023, the centre launched CLASSLA-web , a new collection of large web corpora for Slovenian, Croatian and Serbian, which can be queried using the CLARIN.SI concordancers (noSketch engine). See this tutorial to learn how to query these corpora.
The Netherlands
👉 PRO-TIP: For examples of information sheets and consent forms for collecting speech data from children, download the templates from the DELAD website.
Switzerland
👉 PRO-TIP: Please remember that even if a country is not a member of CLARIN, teachers, students and researchers can still benefit from all the resources and tools hosted by other national repositories that are publicly available. |
To summarise this section, teachers can use the CLARIN local networks to:
- Contact the CLARIN National Coordinator and/or national helpdesks to learn more about what CLARIN has to offer in a specific country;
- Contact a K-centre to find support for a university course/programme, check for training opportunities and seek guidance in getting access to the resources and tools in their country;
- Apply for a mobility grant to visit a centre or set up a teacher exchange and training programme;
- Search for other funding opportunities, e.g. to organise events or train-the-trainer workshops.
👉 PRO-TIP: More information about the CLARIN K-Centres is available in Tour de CLARIN and Impact Stories, which showcase innovative research and educational projects. |
“The integration of infrastructures into teaching should be seen more as a journey, rather than a goal in itself.” (Vesna Lušiky, Lecturer in Translation Studies, University of Vienna)
When creating a research-based course in linguistics and language-related disciplines, extensive planning and research are necessary to ensure that the course meets students’ needs and achieves desired learning outcomes. It is crucial to access research infrastructures, repositories, and language resources that teachers and students can freely use to collect, process, analyse, and deposit language data in the classroom. Free resources and tools can be used to design an engaging, and practical hands-on curriculum.
This part of our guide showcases how CLARIN core services, collections of open corpora and online natural processing tools can be used to enhance the teaching of language data discovery, analysis, and archiving skills. As corpora are one of CLARIN’s most valuable language resources, we begin with general recommendations for teachers who consider integrating data-driven learning and corpus-based pedagogy in language-related disciplines. We continue with a brief overview of the CLARIN central services for data discovery, analysis and processing, which are presented in more detail through quick step-by-step guides and references to additional teaching and learning content on Moodle. Finally, we show how to find natural language processing tools in the infrastructure that are often used or may be suitable to explore in educational settings.
The insights collected from the lecturers participating in the UPSKILLS events revealed that the successful implementation of infrastructures, language resources and tools in linguistics and language-related programmes depends on several factors:
-
- Teachers’ own perception, attitude, and confidence in applying data-driven learning and corpus technology tools in the classroom;
- Students’ background and their study load;
- The flexibility of the curriculum.
Hence, this section provides general recommendations on how teachers can improve their perception and skills in applying corpus-based pedagogy and technologies in the classroom through the CLARIN knowledge infrastructure and wider linguistic community. Teaching assistants and trainers who never used corpora in teaching may find this section useful.
One of CLARIN’s most essential language resources are corpora of various types, modalities and languages. Corpora are often used to answer research questions in both social sciences and humanities domains (McCarthy & O’Keefe, 2010), and in data-driven learning (DDL; Johns, 1991) to encourage students to analyse corpus data independently using corpus query platforms, create hypotheses, identify linguistic patterns and formulate rules, and verify the validity of grammatical rules. DDL can benefit, for example, foreign language learners by providing them with access to corpora containing authentic texts and user-friendly tools to explore linguistic patterns and trends in language use and reach their own conclusions (Bernardini, 2002; Boulton, 2009 & 2017). This approach gives students autonomy over their learning process, helps them develop critical thinking skills and turns them into “researchers”. According to the literature (Boulton 2009; Gilquin & Granger, 2010), data-driven learning based on corpora has not yet been widely adopted in some language-related programmes because teachers are often unaware of the benefits of using corpora for pedagogical purposes. Second, teachers need a high level of corpus literacy (Mukherjee, 2006) to be able to develop corpus-based pedagogy (CBP), “the ability to integrate corpus linguistics technology into classroom language pedagogy to facilitate language teaching’’ (Ma et al., 2021, p 2). Furthermore, teachers need to learn to switch to a “less central role’’ in the classroom than in traditional teaching and guide the students through the learning process (Gilquin and Granger, 2010). Finally, DDL may not suit all learner types because it requires certain technical expertise to work with the corpus technologies. It can also be time-consuming, as learners need to analyse concordance results and draw their own conclusions. While students appreciate the use of corpus concordancers in the translation classroom, it may take a long time to teach them how to collect enough evidence to be able to make generalisations. Students may also find it difficult to understand how to transform knowledge from another language to another, connect several language resources (e.g. WordNet vs Valency Lexicon), apply linguistic tests, and make decisions regarding translation equivalents. (Petya Osenova, Professor and Researcher in Syntax, Morphology and Corpus Linguistics, Faculty of Slavonic Languages, St. Kl. Ohridski University, Sofia, Bulgaria) Teachers who never used corpora and corpus technologies in the classroom are recommended to first delve into the literature to understand whether incorporating corpus-based pedagogy (CBP) and data-driven learning in their curriculum would benefit their specific linguistic sub-discipline, teaching style and the students’ background, level and learning style. A good literature review of how corpora are used as a pedagogical tool in various areas of linguistics, see “Part III. Corpora, language pedagogy and language acquisition” in the Routledge Handbook of Corpus Linguistics (O'Keeffe & McCarthy, 2022). Other scenarios in which corpora are used in educational settings are teaching corpus linguistics as an academic subject, using corpora to inform syllabus design and development of educational resources (e.g. dictionaries and grammars) and involving students in the development of language resources (collect, design, and compile corpora) (Cheng & Lam, 2022). Finally, students can also be taught how to share and archive a corpus at the end of the project, solving all the issues related to handling personal and sensitive data. See Section 6 in the Moodle UPSKILLS learning content, Introduction to Language Data: Standards and Repositories. References: Before integrating corpora or any other language resource or tools in the classroom, teachers should first gain some hands-on experience themselves. For example, learn basic methods in corpus linguistics, different types of corpora and corpus technologies, how to extract relevant data from corpora, count occurrences of phenomena, and do statistical analyses (Lin 2019). Some of the active learning content developed in UPSKILLS and available on Moodle can be a good starting point: Besides proficient use of corpora and corpus technology, teachers will also need pedagogical knowledge to design corpus-based activities and assessments that match their course's overall goals and students' learning needs (Ma et al., 2021). As practice shows, research is often prioritised over teaching, with lecturers having excellent research skills but poor pedagogical skills (van Dijk et al., 2020). Below, we recommend established workshops, summer schools and free online courses, which could help teachers build up both their technical and pedagogical skills: Finally, we also recommend keeping an eye on the upcoming CLARIN workshops, which aim to educate and train various stakeholders on the use of corpora and other technologies. References: Sometimes, classes in Applied Linguistics, such as Corpus Linguistics and Computational Linguistics, and Translation Studies consist of students with mixed backgrounds (language and humanities vs. computer scientists) and coming from different countries. Such classes pose additional challenges in teaching and learning because the teacher needs to find ways to motivate language and humanities students to work with computational methods and tools. In contrast, the computer scientists need to learn about linguistics. Furthermore, access to multilingual language data repositories and resources is needed to design learning activities in the students’ preferred languages. For example, the Virtual Language Observatory and CLARIN Resource Families may be useful in multilingual language technology classes because they provide access to different types of corpora and datasets in multiple languages. Therefore, it is important to identify students’ backgrounds, languages, technical skills, research interests and their level of interest in technology to be able to tailor the language resources and research methods chosen for the classroom. If the learning activities cater to the needs of the students’ linguistic concerns or research interests, they will feel more motivated to engage with technology. Whenever possible, classes should be tailored to certain student groups instead of attempting to meet the needs of a varied group of students in each course (Baldridge & Erk, 2008). Try to split the RBT course into feasible portions with clear learning outcomes and consider students’ own interests in making the connections between linguistics and the more technical parts (e.g. programming in Python, data handling). You could start with the linguistic questions, then use technical knowledge to address the questions and translate the answers back into the research domain. (Louis ten Bosch, Associate Professor of Deep Learning and Automatic Speech Recognition, Radboud University, the Netherlands) References: After consolidating their knowledge about scientific research and corpus linguistics, teachers are invited to use this guide and the accompanying course on Moodle, Introduction to Language Data Standards and Repositories, to get acquainted with the CLARIN central services and identify those language resources and tools suitable to include in teaching. The exploration may be easier if it is based on a clear research question, study or project that teachers intend to formulate in the classroom. Consider the type of data the corpus should have, register, languages, size, availability, the time period that the corpus covers, etc. When testing and selecting corpus technology tools, it is recommended to test them properly to identify those functions that will help achieve a specific goal in the classroom. The final choices and implementation of language resources and tools in the classroom are often influenced by teachers’ own perceptions, attitudes, and confidence in applying corpus technology in the classroom (Leńko-Szymańska, A., 2017; Ma et al., 2022). References: Once appropriate resources and tools have been identified for classroom use, the learning outcomes of the course should be adapted in order to target practical skills related to the use of infrastructure, create and curate learning materials and design corpus-based activities and assessments. To save time and effort, teachers are recommended to pick and choose units of individual learning activities from the UPSLILLS Moodle platform, build on them and share them with their students. Instructions on how to export and reuse learning content are included within each learning block. When creating learning content for technical-oriented tasks and using different tools, remember that tools change rapidly, so updating the content will require time and effort. Therefore, to save time, consider reusing tutorials provided by the infrastructure and tool providers, and adapting them to match the students’ learning objectives and levels. If learning materials need to be created from scratch, the focus should be on making them as modular as possible so that other teachers can easily update and reuse them. Moreover, in multidisciplinary courses, learners come from different fields and backgrounds, and some may not yet have all the required skills. This can place an extra burden on the teacher. In online teaching, the issue might be partially solved by creating small, self-contained and well-described learning objects that can be used flexibly in many different courses and replaced or removed when the content becomes obsolete. Effective planning is crucial when introducing corpora in the classroom. We have compiled a general framework of helpful teaching strategies from Sripicharn (2010), Tribble (2010), and Lessard-Clouston & Chang (2014) that can be referenced when starting to teach with corpora regardless of your linguistic sub-discipline. After students have increased their corpus literacy skills, they can be introduced to data-driven learning by challenging them to work on a small-scale research project and answer a specific research question. Bennett (2010) proposes a general framework for using corpora in language teaching, similar to the one above but starting from a research question. According to lecturers’ testimonials in Simonovic et al. (2023), using technology in the classroom (even when starting from a simple task, such as organising data in an Excel file) can disrupt students’ learning experience when unexpected technical issues arise. Students will need guidance and support throughout the course. For example, more advanced students may be affected if the lecturer needs to invest more time in guiding those students who lack basic scientific knowledge and technical skills. A more interdisciplinary approach to teaching language and linguistics could help tackle such issues. For example, students could be recommended to follow computer science courses, while teachers could create joint projects and assignments to ease their workload. However, if such an interdisciplinary approach is not possible in your programme, here are a few practical tips. Tool handouts, video tutorials, and a pre-defined folder structure for file organisation can help minimise technical disruptions during class. Collaborative online environments like Google Drive, Colab, GitHub, or Open Science Forum (OSF), containing all the files and instructions for the class, can help increase teaching efficiency and minimise disruptions. Additionally, creating a forum within the learning management system (such as Moodle or Blackboard) can assist in reducing the teacher's workload by allowing students to interact with their peers and ask questions about any technical difficulties they may face while completing homework or class assignments. This approach promotes independent problem-solving skills among students and only requires teacher intervention when necessary. References: Undertaking and monitoring research projects involving language resources can be challenging and require continuous guidance and feedback to help students improve their work. With many changes that may occur during the research process, it is easy to get lost and confused. However, keeping track of information at different stages can help clarify ideas, resulting in faster progress. In line with this, our consortium partners from the University of Zurich have developed an interactive research tracking tool that enables students to track their progress during projects. Students fill in the template using the student version of the tool. Teachers then use the teacher's version of the tool to provide brief feedback in the designated field. At the project's onset, it is up to the teachers and students to agree on the reporting frequency. Each submitted report should receive short feedback from the teacher. The preferred sharing mode (email, online drive, etc.) will also be decided. The research tracker can be downloaded from the landing page of this guide. References: Language resources and technologies can support teaching and research across various disciplines to teach students how to develop a data-driven mindset and draw insights and conclusions from large volumes of text. While testing and using various language resources and tools corpus classroom use, collecting feedback from students on the usability of the resources, tools and infrastructure and their usefulness in educational settings is beneficial. For example, Lusicky and Wissik (2016) evaluated the usability of language resources like corpora and translation memories, disseminated through research data catalogues such as Virtual Language Observatory, Meta-Share, and ELRA, for translation studies scholars and students. According to the authors, repositories can help researchers make their language resources more FAIR and meaningful for translation studies by including specific metadata catering to the field. For instance, in the case of parallel corpora, it is essential to know the original language, the reliability of the source texts, whether the translation was obtained via post-editing machine-translation output, as well as the names of the translators and their native languages. The following criteria could be used to evaluate your own experience with the tools and also collect insights from students. All the feedback, suggestions for improvements or additional features could then be shared with the infrastructure and tools developers to improve the subsequent versions of their tools. See the testimonials below as an example: The language resources available via the CLARIN infrastructure are very important for my teaching. It is vital that the same materials are persistently available and that they can be cited consistently. I try to teach first-year students to search for corpora and other resources that can be relevant to them via CLARIN platforms, and the more advanced students can benefit from tools, good practices and guidelines that help them to process and make available the resources they create. Praat and ELAN have been around for a long time, and teachers can be confident they will remain accessible in the coming years. University students need good examples of reproducibility, scientific references and citation practices for tools and data since these will be the building blocks they will work with in the future. (Mietta Lennes, Lecturer of Speech Technologies, University of Helsinki) The students perceive corpora as something entirely new, they tend to get scared initially and need some time to familiarise themselves with different types of queries, and they tend to struggle with regex. But these challenges can be overcome. (Anonymous lecturer, UPSKILLS Questionnaire of Lecturers) References:blank
1. Explore best practices in data-driven learning & corpus pedagogy
2. Build up your corpus literacy and pedagogical knowledge
3. Know your students
4. Identify and select language resources and tools
5. Curate, adapt or create learning content
6. Teaching in the classroom
👉 PRO-TIP: A good overview of corpus-based activities per linguistic sub-discipline is available in the Routledge Handbook of Corpus Linguistics (O'Keeffe & McCarthy 2022).
👉 PRO-TIP: See the student projects that the UPSKILLS consortium partners and CLARIN jointly developed in the Processing Texts and Corpora and Introduction to Language Data: Standards and Repositories on Moodle.
7. Tracking research projects
To learn more about student project design and reporting formats, please see the Guidelines for the Students' Projects and Research Reporting Formats (Simonović, M. et al., 2023).
8. Evaluate and share your experience
Teachers can use the CLARIN central services in the classroom to teach language data discovery, (re)use, sharing, citing and archiving.
-
- Use the Virtual Language Observatory (VLO) to search for full-text language resources of different types, languages, modalities, time periods, formats and licences.
- Find a matching natural language processing tool via Language Resource Switchboard (LRS) to process language resources or texts and perform more advanced linguistics tasks, such as different types of automatic annotation, morphological analysis, distant reading, terminology and keywords extractions, topic modelling, etc.
- Collect the resources discovered in the VLO or any other research data repository in a virtual collection in the Virtual Collection Registry (VCR) that can be cited and shared with other teachers or students. This service allows users to save resources for later exploration and processing. In contrast with Zotero or Zenodo repositories, the VCR allows you to add multiple resources to a collection and cite the entire collection using persistent identifiers, such as handle or DOI.
- Use the Federated Content Search to search for specific linguistic patterns across several collections of corpora in several repositories simultaneously. You can use CQL for queries and download the search results in different formats.
- Search for corpora for specific registers and languages in the Language Resource Families. Most corpora are freely available, can be cited, and downloaded from the repository where it is located. Some corpora are directly available for query in online concordancers, such as KonText, Korp and NoSketchEngine.
- Language resources created collaboratively as part of a research-based project or in the context of a thesis can be deposited, shared and archived through a suitable CLARIN repository. The repositories adhere to the FAIR guiding principles for research data management and sharing. See the depositing services for general depositing guidelines and an overview of the centres providing support in this process.
If you have never used CLARIN before, watch the video below to learn how the Virtual Language Observatory and the Language Resource Switchboard are integrated to enable language resource discovery and reuse for research purposes:
👉 PRO-TIP: To help you evaluate the suitability of the CLARIN infrastructure (or any other infrastructure) for teaching language and linguistic research, we recommend first exploring and testing the core services, some tools and language resources yourself with the help of this guide and accompanying learning content on Moodle, Introduction to Language Data: Standards and Repositories, especially Unit 3: Finding and (Re) Using Language Resources in CLARIN Repositories. |
This section presents the CLARIN central services, which teachers and students can use in the planning and data collection phases of a research project to search and locate language resources that can help answer specific research questions, replicate a dataset, build a corpus, or train a language model. The central services for data discovery are the Virtual Language Observatory, Federated Content Search, Resource Families, and the Virtual Collection Registry. While many language resources are accessible through CLARIN, we will mainly demonstrate how to search, locate and use corpora of different types, languages and modalities.
After getting acquainted with the basic functionalities of each service and understanding what they can be used for, try to test it by formulating a research question for a specific register and language (s). This would make the searches more focused and identifying appropriate corpora and tools easier.
📖 Teaching and Learning Resources on Moodle
|
The Virtual Language Observatory (VLO) central catalogue automatically harvests metadata on language resources contributed by researchers in CLARIN member and observer countries. It offers advanced search functionalities that facilitate the easy discovery of language resources, such as corpora, lexica, grammars, multimedia recordings, digitised texts such as books, articles, and transcripts of parliamentary debates, software & web applications, and even training materials.
Faceted Search in the VLO
Because of the large amount of data, there are multiple ways of exploring the VLO, e.g., full-text search, facet browsing, or geographic overlay. The advanced filters can help narrow down the search results and find resources or text collections in a specific language, resource type (text, audio, dataset, corpus, software, video etc.), modality (spoken, writing), format (text, audio, image, specific keywords), temporal coverage or availability (for public, academic, restricted use).
When searching for resources, remember that the search results might contain duplicate entries, incorrect or incomplete titles/descriptions. The flag icon on the top right corner can be used to report issues via the VLO feedback form. The service is continuously improved to facilitate easy data discovery.
Each resource in the VLO can be accessed directly via the unique/persistent identifier, i.e.handle, pointing to the landing page of the repository where the resource creator initially deposited the resource. Teach the students to use this handle to reference the landing page online and in their publications. CLARIN endorses the Data Citation Principles.
👉 PRO-TIP: If you are unfamiliar with the current practices in citing language data, see Unit 4 of our Introduction to Language Data: Standards and Repositories on Moodle: Citing Language and Linguistic Data. |
Assuming you are searching for a digitalised text collection of European treaties to build a corpus, go to the VLO and perform the steps described in the quick guide below. Was it easy to use, find and access the Treaty of European Union collection?
Searching for Language Data in the Virtual Language Observatory
When you find a language resource in the VLO that might be interesting to explore for research or teaching, carefully read and interpret the metadata fields used to describe the resource, especially the record details, links and their availability. Each resource is described based on metadata standards (e.g. CMDI, Dublin Core, OLAC), which provide helpful information about who created the resource and how. It is also advisable to review the metadata of the resource from the perspective of your specific linguistic sub-discipline. For instance, a study conducted by Lusicky & Wissik (2016), mentioned earlier, assessed the usability of language resources such as corpora, translation memories, terminology resources, and lexica from the VLO and other research data repositories (META-SHARE, ELRA) for translation studies scholars and students.
Further, the VLO records contain information about the licence assigned to the resource and terms and conditions of use. Although CLARIN advocates for open science and access, data creators may restrict access to data collections due to sensitive data. It is important to note that resources and tools in CLARIN are assigned either a public (PUB), academic (ACA) or restricted (RES) licence. Public resources can be reused without copyright restrictions, whereas academic or restricted resources can only be used under specific conditions.
Finally, the digital text collections in the VLO can be processed and analysed with integrated Natural Processing Tools. For example, suppose you find a resource in the VLO in plain text format. You can process it directly using one of the Language Resource Switchboard tools, e.g. use WebLicht to annotate the plain text file automatically, use UDPipe to produce syntax trees or use the LINDAT machine translation service to translate the file into another language. You will learn more about Switchboard in Section 4.2. Data Processing and Analysis. If you do not want to process the file immediately, you can choose to queue it for submission to a Virtual Collection. The following figure shows how to access the Switchboard and Virtual Collection Registry directly from the Links area of the VLO record.
Process the text file with Switchboard or send it to a Virtual Collection
The metadata quality of language resources and their suitability for educational settings can be evaluated through a simple checklist, e.g.,
-
- Does the language resource provide sufficient metadata to help you decide to use it in your research and/or teaching? If used for research purposes, does it sufficiently address the research problems?
- Who created the resource, and when?
- Is it reliable? How often has the resource been viewed and/or downloaded by others (researchers, teachers, students)?
- What type of language data has the resource creator collected, and from whom, when and where? How was the data collected and processed?
- Does the resource creator provide any information about how the resource can be used in either research or teaching?
- In what format the data and metadata are available? Are the files in the resource available in a common format compatible with other tools?
- Does the repository provider offer an online service to help you look inside the language resources/ dataset?
- If the infrastructure does not provide any tools to preview or explore the contents of the resource, can you and your students download it and use it for processing and analysis in other tools without restrictions?
- Do you and your students need special software skills to process and analyse the dataset? If you lack the skills to process a large dataset, you can try to contact the data provider or the research data management department at your university and ask for help.
After the students learn to evaluate resources and their metadata properly, teach them how to use them in the tools available through the infrastructures or other preferred linguistic tools.
📖 Teaching and Learning Resources on Moodle Introduction to Language Data: Standards and Repositories
|
References:
- Andreassen, H. (2019, March 04). The acquisition of definiteness: Analysis of child language data. OER Commons.
- Lušicky, V., & T. Wissik, T. (2016). Evaluation of CLARIN services, user requirements, usability, VLO, and translation studies. In Selected Papers from the CLARIN Annual Conference 2016, Aix-en-Provence, France, pp. 63-75. Linköping University Electronic Press, Linköpings universitet.
While the VLO allows only metadata searches to locate full language resources and texts, one can use the Federated Content Search (FCS) to identify specific linguistic patterns (e.g. collocations) across various corpora hosted in different CLARIN centres simultaneously. The corpora stay at the centre where they are hosted; therefore, the underlying technique is called federated content search. As of April 2023, there are 207 corpora searchable via FCS in various languages.
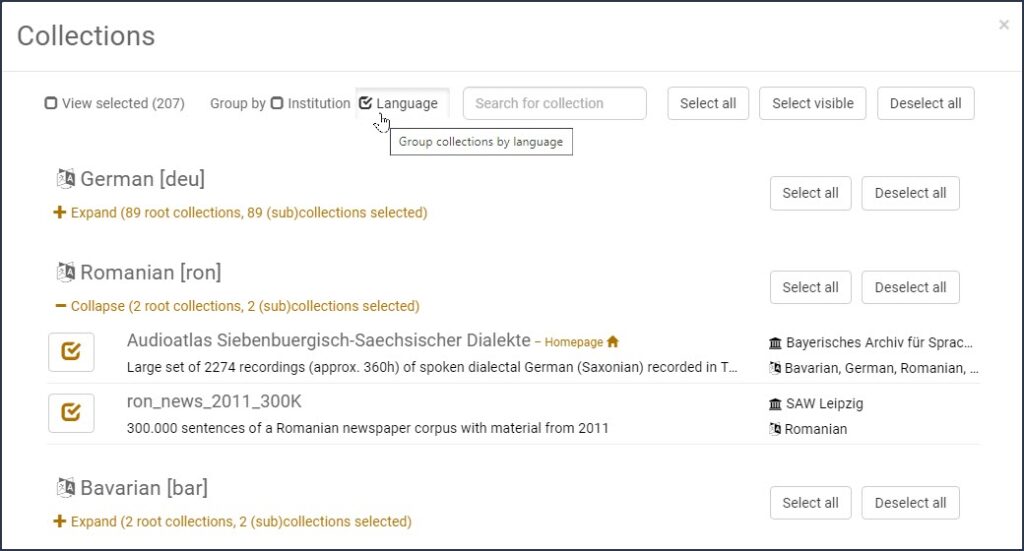
Collections of corpora browsable in Content Search sorted by language
To view the available corpora per language, go to the Content Search main interface and click on Collections. Then, tick the Language box to group the collections per language, as in the figure above. Finally, click the + sign to expand and view the corpora available for a specific language. Monolingual searches can be performed with the help of integrated Contextual Query Language (CQL) queries. The search results can be displayed as Keywords in Context and downloaded in various file formats.
Use the steps described in the following quick guide to learn how to use the FCS service. You can, for example, search for collocations in a specific language and export the results.
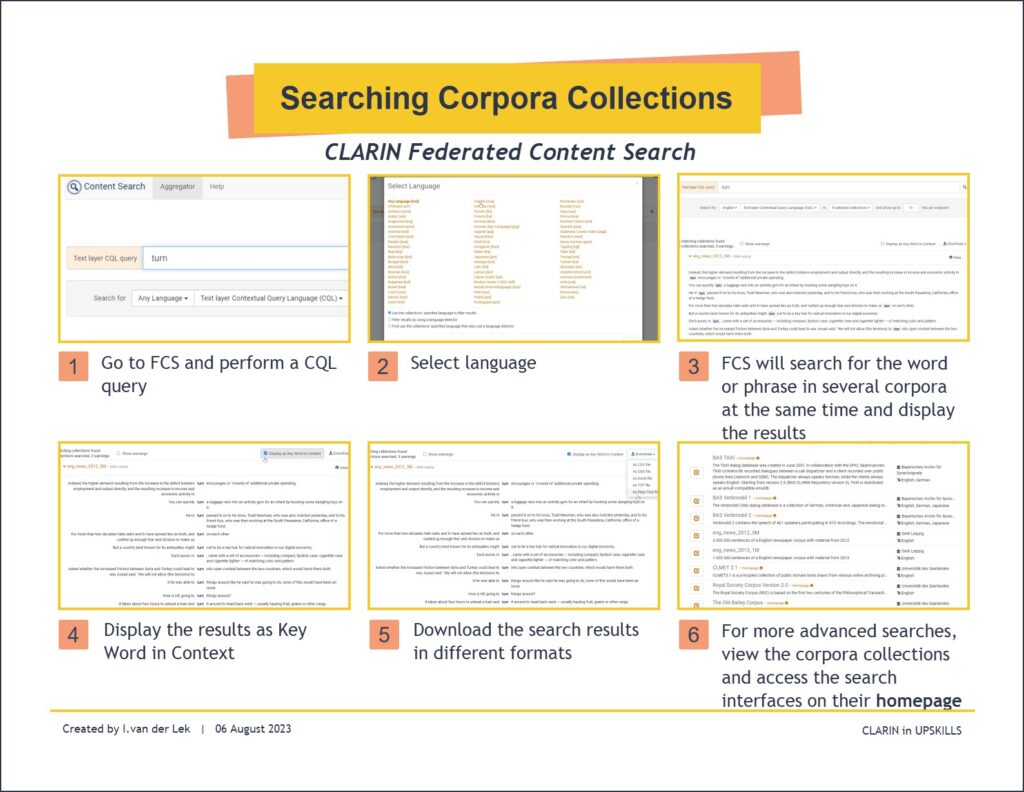
Searching corpora collections through Federated Content Search
👉 PRO-TIP: Although the service offers basic functionalities, it can help teach students basic corpus query searches to investigate how certain words and phrases are used in context. To perform more sophisticated queries, view the collections of corpora available through the FCS service and go to the search interface of the centre hosting the text collection. |
Research has demonstrated that scholars, instructors, and learners have used corpora for “data-driven learning” (Bernardini, 2006) to examine genuine language usage, contextual subtleties, and actual linguistic variations, allowing them to make generalisations about language use. Teachers who already use corpora in their own research and/or teaching methods may already have one or more preferred corpora and corpus analysis tools they are accustomed to using in the classroom.
For those considering to integrate corpora into their course or programme, the CLARIN Resource Families can be an invaluable starting point. The families include various corpus types (and tools), each catering to specific linguistic or research needs (see below image), and are meant to facilitate comparative research. Additionally, the listings in each resource family are sorted by language and provide brief overviews about the size of the resource, text sources, data type, time periods, annotation types, standards, formats and licence information. Unlike the Virtual Language Observatory, this overview of corpora collections is much user-friendlier and makes it easier to identify what type of corpora matches the goals of your course/project/assignment.
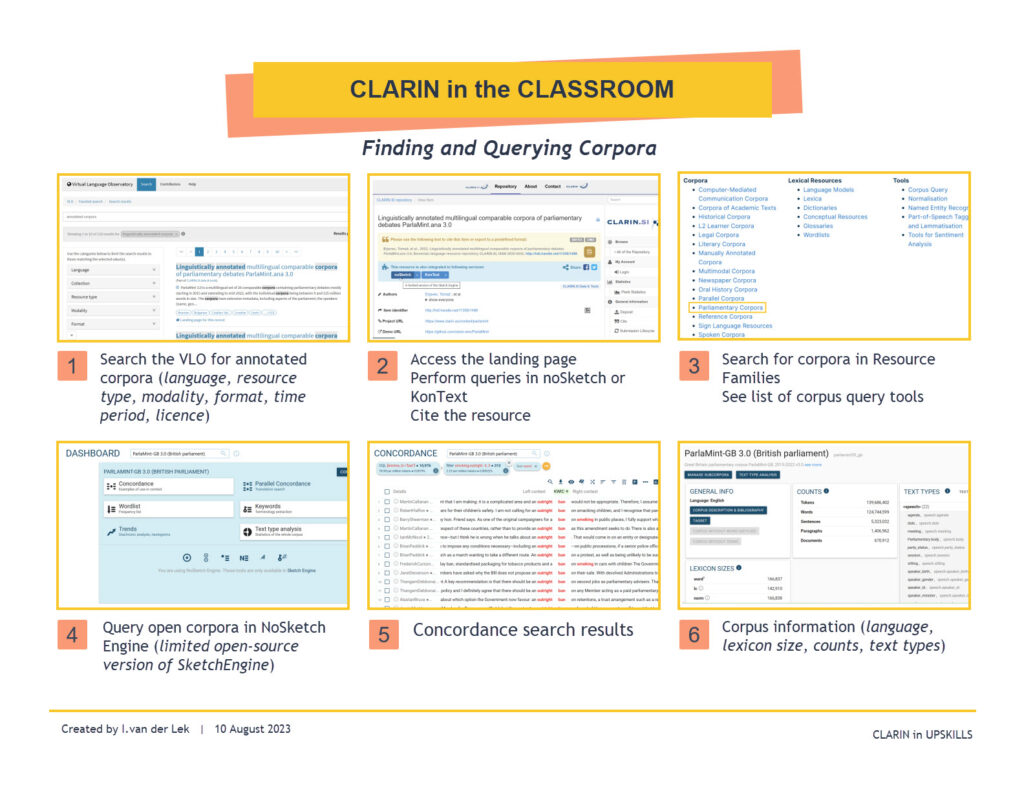
Finding and Querying Corpora
👉 PRO-TIP: If this is your first time working with corpora, see A Practical Handbook of Corpus Linguistics by Paquot & Gries (2020) to understand how different corpus types can be designed, analysed and compiled (see chapters 1 to 3). The handbook can also be used as a course book in the classroom or for individual study. |
Most importantly, many corpora listed in the resource families are available in open access and can be directly queried in online concordancers, such as Korp, Corpuscle, KonText and noSketchEngine. These concordancers are integrated into the infrastructures of the CLARIN national repositories in Finland, Norway, the Czech Republic and Slovenia, giving access to many corpora developed by researchers in their communities. Moreover, some corpora come with detailed tutorials demonstrating how to use them for research. The tutorials are available in open access, and you can refer the students to follow them independently or adapt them for classroom use. All these aspects make the resource families of corpora a great open educational resource, which can be a valuable addition to the teachers’ toolbox, especially in the era of hybrid learning and teaching.
Nevertheless, several factors can make locating an appropriate corpus for educational purposes challenging. First, as Deshors (2021) points out, different corpora serve different purposes. While some specialised corpora are more suitable for research (e.g. Louvain International Database of Spoken English), others are used as teaching/learning resources (e.g. BNC, COCA). On the other hand, while larger corpora may provide more comprehensive coverage, more specialised corpora may be more suitable to answer more specific research questions. Second, the design and accessibility of a corpus may pose challenges for both teachers and learners.
For example, some studies show that Lextutor, a text-based concordancer, may be too technical for learners. In contrast, AntConc has been found suitable only for adult learners with a high level of English proficiency. Other corpus query platforms, such as SketchEngine, are powerful and user-friendly but available only via subscription, which could be a financial barrier for some universities. Finally, learning how to effectively use corpora in the classroom and design corpus-based materials to fit specific teaching objectives and students’ levels of digital literacy can be challenging and time-consuming.
To help with these challenges, we have gathered several samples of CLARIN corpora and tools currently used in teaching and training by UPSKILLS consortium partners and other educators within the CLARIN community. Furthermore, we have provided links to available tutorials that can be repurposed for classroom use.
If you teach language variation or pragmatics, you may be interested in using computer-mediated communication corpora (CMC) in the classroom because they include informal writing styles. The resource family of CMC corpora contains open CMC corpora in Slovenian, Czech, Dutch, Estonian, Finnish, French, German, Italian, and Lithuanian. Most corpora are tagged and available for exploration via integrated concordancers. If you are searching for multilingual CMC corpora, go to resource families and search for the following corpora: sms4science and What’s Up, Switzerland? . Both corpora have been compiled by Swiss researchers in the Swiss official languages and their varieties and are freely available for linguistic research through online corpus query platforms. The platforms allow you to perform simple and advanced queries, produce a frequency list and export the results. The corpora are also a great example of collecting, processing, cleaning, anonymising and annotating CMC corpora. Another example of a well-documented CMC corpus is the Twitter corpus Janes-Tweet 1.0, a corpus of Slovenian tweets collected between 2013 and 2017. This corpus is tokenised, and it can be queried both through NoSketch Engine and the KonText concordancers. Moreover, the corpus is published under a CC BY-NC 4.0 licence and can be downloaded from the CLARIN.SI repository. To learn how to use this corpus for research, see the PARTHENOS tutorial, Using social media corpora in CLARIN. 👉 PRO-TIP: If you or your students are interested in using CMC corpora for research purposes, we recommend the following learning resources: Learner corpora are a valuable pedagogical tool for the teacher-researcher because they contain spoken and/or written texts produced by language learners. As per August 2023, the CLARIN resource family of L2 learner corpora contain 72 L2 corpora, out of which 11 are multilingual, while the rest are monolingual forms and various modalities (written, spoken, video). If you are teaching English as a Foreign Language (EFL), you may already use BAWE, BNC and COCA in your classroom. Here we would like to highlight a few corpora and tools that provide useful pedagogical materials for teachers. The British Academic Written English Corpus (BAWE) is often used to teach linguistic analysis of different genres and academic writing registers, helping students understand academic conventions and develop writing skills. The corpus is hosted in the Oxford Text Archive Repository, and discoverable via the Virtual Language Observatory. Academic users can use it free of charge for academic purposes, and query it in Sketch Engine or Lextutor. To learn how to use it in SketchEngine, see the detailed Using SketchEngine with BAWE tutorial on the website, which consists of 7 lessons demonstrating how to make a simple concordance search, analyse collocations, learn how to use corpus query language and extract keywords. To make it easier for teachers and learners to use BAWE in the classroom, a collection of quicklinks to concordances in SketchEngine have been directly integrated as feedback directly in the students’ assignments to help them improve their academic writing errors. This is a great example of how corpora and data-driven learning (DDL) are used to improve learner autonomy. The Das Digitale Wörterbuch der Deutschen Sprache (DWDS = Digital Dictionary of the German Language) corpora, developed at the CLARIN-D centre Berlin-Bradenburg Academy of Sciences and Humanities, are used at the University of Kansas as an open educational resource to teach German to English-speaking learners (Vyatkina, 2020a). The resource contains a guide to the main search functionalities of DWDS and a set of interactive corpus-based activities created in h5p. The learning activities have been designed based on guided induction, combining data-driven exploration with linguistic expertise to analyse the DWDS corpus. This pedagogical resource is a great example of how large open-source corpora in languages other than English can be integrated into the classroom (see Vyatkina, 2020b, for more details). Another tool worth mentioning here, although it is not a CLARIN tool, is SkELL. This tool is a free online version of SketchEngine specifically developed for English language learners and provides a very simple search interface to look up words and their meanings in a one-billion-word web corpus. The tool includes a concordance tool, word sketches and a thesaurus functionality. The concordance results are presented in a sentence format. Teachers can use SkeLL to create exercises (gap-fill, matching and multiple choice) to teach students how to infer the meaning of words and phrases, analyse synonyms/polysemy, frequency, word association etc. For more examples of learning activities using SketchEngine and SkeLL, see Thomas (2017). References: Comparable corpora, defined as “a collection of texts composed independently in the respective languages and put together based on similarity of content, domain and communicative function” (Zanettin 1998:614), have extensively been used in educational settings. In translation teaching, for example, they are used as a pedagogical tool to help students enhance their understanding of the source language text and produce fluent translations ParlaMint is one of the most well-documented multilingual comparable corpora in CLARIN. It comprises 33 parliamentary corpora that cover most of the EU languages. These corpora are an important multidisciplinary language resource for social sciences and humanities researchers. They can be accessed through online corpus query platforms and are available in many languages. This makes it easy to integrate parliamentary corpora into classroom teaching. For example, search in Parliamentary Corpora Resource Family for ParlaMint.ana 3.0 (Erjavec et al., 2023). This collection contains a multilingual 26 comparable corpora of parliamentary debates, with each corpus being between 9 and 125 million words in size. What makes ParlaMint dataset easy to use both for research and teaching is that the corpora are tokenised and syntactically parsed using the Universal Dependencies (UD) framework, and annotated with named entities, which enhances the understanding and analysis of the data. The corpora are fully open and available through the noSketch Engine concordancer, a user-friendly tool to introduce the students to concepts in corpus linguistics analysis. If you prefer to use the corpus in another concordancer, you can download it (with or without linguistic markup) from the CLARIN.SI repository. The corpora have been used in several editions of the Helsinki Digital Humanities Hackathon (Semparl - Cities in parliamint; Parliamentary Debates in COVID Times), various tutorials, university courses and student theses; see the examples below and more information on the ParlaMint project information page. Here we would like to highlight two tutorials demonstrating the use of the parliament corpora in research, which teachers can integrate into any course or programme involving modern languages, digital humanities, social sciences and corpus linguistics. Both tutorials are reused under a CC-BY-NC-ND 4.0 licence, which means that you can adopt and integrate the tutorials in your classroom, giving appropriate credit, but you are not allowed to use the material commercially. You may not distribute the materials further if you modify and build upon them. The ORVELIT corpus is a comparable monolingual corpus of original and translated Lithuanian consisting of four sub-corpora of original and translated fiction and popular science. Although the corpus is not available through a concordancer, it can be downloaded from the CLARIN-LT repository (both in a raw and morphologically annotated version). The corpus is being used in the curriculum of the MA course in “Contrastive Stylistics” at Vytautas Magnus University. Students get acquainted with building procedures and characteristics of the ORVELIT corpus, after which they are encouraged to think of possible research questions and registers that would be interesting to explore using the corpus. In addition, students learn to create a basic research data management plan. Other learning activities include downloading the morphologically annotated and raw versions of the corpus to investigate the features of original and translated Lithuanian independently with the help of a corpus analysis tool. Finally, students learn to generate and compare wordlists, keyword lists and concordances and discuss their findings on the similarities and differences between original and translated texts. The ORVELIT corpus has been used to design learning activities about the use of Lithuanian collocations in teaching, learning and translation in this online resource book: → Kovalevskaitė J., Rimkutė E., Vaičenonienė J. 2022: Lietuvių kalbos kolokacijos: vartojimas, mokymas(is) ir vertimas (Lithuanian Collocations: Usage, Teaching, Learning, and Translation). Kaunas: Vytauto Didžiojo universitetas. ISBN 978-609-467-524-9, https://doi.org/10.7220/9786094675249. (The activities in the book have been designed using many other Lithuanian language resources in the VLO, which are all listed in the tutorial entry on the CLARIN Learning Hub.) References: Parallel corpora are defined as a “collection of texts in language A and of their correspondent translations into language B” (Baker, 1995). They can be bilingual or multilingual and contain published translations. Due to the lack of parallel texts, parallel corpora are challenging to build and they are smaller than the monolingual or comparable corpora. For a discussion of issues and challenges in compiling and analysing parallel corpora, see Lefer M.-A. (2020). Parallel corpora are often used in corpus-based contrastive linguistics and translation studies (Levishina, 2016). In translation studies, bilingual concordances can be used to analyse cross-linguistic correspondences between lexical items, syntactic patterns or grammatical structures and discuss translation strategies for proper names and culture-specific elements (Lefer M.-A.,2020, p.263). In the practical translation classroom, parallel corpora are also used to teach students how to import it into their computer-aided translation tool and use it as a translation memory (e.g. using corpora from OPUS) or extract bilingual terms using such tools as Synchrotem to create bilingual glossaries. Furthermore, parallel corpora are used in the machine translation/AI classroom to teach students how to train statistical machine translation engines. Last but not least, Bluemel, B. (2014) showed that parallel corpora can have a pedagogical value in foreign language learning provided the corpus is specifically designed for use in the classroom. He developed a Chinese/English parallel corpus to help high-school students improve their reading comprehension and writing skills. The CLARIN resource family provides access to 87 parallel corpora, out of which 5 contain language data in more than 50 languages. Here, we highlight those that can be easily integrated into the classroom. Well-known parallel corpora used in corpus-based translation/contrastive studies and NLP are: EuroParl, DGT-Translation Memory, European Central Bank parallel corpus, Opus, Helsinki, ParaCrawl. You can view the most important metadata information in the CLARIN Resource Family overview. InterCorp, a parallel corpus of more than 40 languages, is often used for comparative research in foreign language teaching, translation studies, theoretical studies, and NLP (Čermák 2019). The texts come from various sources, such as fiction, EU legal texts, film subtitles, and the Bible. The corpus can be queried via KonText. The Wiki page of the Czech National Corpus provides a nice tutorial consisting of 11 lessons about using KonText and performing queries in a parallel corpus (InterCorp), spoken corpus, diachronic corpus and syntactically annotated corpus. Each lesson contains clear examples of queries and an exercise at the end. Compara is a parallel corpus of English and Portuguese, and it can be queried in a free and user-friendly parallel concordancer. The corpus is used in translation studies, not only for descriptive and empirical research but also by lecturers to prepare exercises and discuss translation problems with students. Unfortunately, few concordancers are available for parallel corpora. In the CLARIN resource family of corpus query tools, we found AntPConc (free desktop-based tool), ParaConc (commercial tool), and SketchEngine (commercial). Teaching and Learning Resources on Moodle: Introduction to Language Data: Standards and Repositories There are numerous parallel corpora available in the CLARIN repositories. To teach students how to use the Virtual Language Observatory to find a parallel corpus for a translation assignment, see References: In June 2023, CLASSLA-web, a new collection of large web corpora (each containing about 2 billion words), was launched. This collection is specifically for Slovenian, Croatian, and Serbian languages, and can be queried using the NoSketch Engine concordancer of CLARIN.SI. To learn how to analyse word usage in contexts, collocations, dictionary examples, and more, visit the tutorial on the CLARIN.SI website. The corpora consist of professional and informal texts, such as forums and blog posts, harvested from the web. This provides linguists and corpus linguists with valuable insights into non-standard language use. Additionally, the monolingual datasets have been linguistically annotated to make them more usable. The previous Bosnian/Croatian/Montenegrin/Serbian Web corpora (srWaC, hrWaC, bsWaC, meWaC) were used in the MA course, From computational linguistics via clinical linguistics to forensic linguistics, at the University of Graz. The corpora can be downloaded from the CLARIN.SI repository and queried in NoSketch Engine and KonText. Swiss-Al: A Multilingual Swiss Web Corpus for Applied Linguistics (Krasselt et al., 2020) contains about 8 million texts in German, French and Italian from selected resources on the web (i.e. news and specialist publications, governmental opinions, and parliamentary records, web sites of political parties, companies, and universities, statements from industry associations and NGOs). Unlike previous web corpora (e.g. WaCky; Baroni et al. 2009), Swiss-Al promotes data-based and data-driven research on societal and political discourses in Switzerland, not for NLP purposes. It can be queried via Swiss-AL Workbench and via CQPweb. 👉 PRO-TIP: If you are interested in more multilingual corpora in other languages, you can find a curated collection on the Wiki of the Association for Computational Linguistics. References: Spoken corpora are compiled as audio and text transcriptions for linguistic purposes, including hypothesis testing and language teaching and for developing grammars and dictionaries. In research, these tools serve various purposes, such as phonetics, conversation analysis, grammar, pragmatics, dialectology, language acquisition, and the creation of acoustic models for speech technology. Additionally, they are used to compile corpora of endangered languages in language documentation. See the Language Archive repository for examples of speech corpora from worldwide languages. According to Gut (2020), compiling and annotating spoken corpora is expensive, time-consuming, and poses numerous ethical challenges for researchers during data collection and dissemination. Therefore, there are scarce and much smaller in size than written corpora. Therefore, Gut calls upon corpus-based linguistic researchers to investigate how existing spoken corpora can be reused in research and teaching. She emphasises that proper reuse is hindered due to lack of documentation, lack of standardisation in annotations and data format, and lack of access and search tools (p.249). As of August 2023, there are about 133 spoken corpora in the CLARIN resource families, mostly monolingual in 15 languages. Some corpora are available for query directly in KonText or Korp. Here we would like to highlight a few spoken corpora used in educational settings. TalkBank is a CLARIN K-Centre offering the world's largest open-access integrated spoken-language data repository. It provides language corpora and audio resources to support researchers in various fields of study, including Linguistics. Academic users can transcribe sound files using the CHAT standard and analyse them in CLAN. A user-friendly guide is available for teachers and students who want to learn how to use CLAN in combination with Praat to analyse speech data. The Slavic data collection from CHILDES corpora is used in the MA, PhD Language Acquisition in Slavic: Obtaining, Representing and Analysing Empirical data in Linguistics course at the University of Graz to help students enhance their research skills in language acquisition, as well as problem-solving and data-analysis skills. The web-based LABLASS platform and Bulgarian LabLing Corpus are included in the curriculum of the linguistic disciplines at Konstantin Preslavsky University of Shumen, Bulgaria. The corpus has been published on the CHILDES platform to facilitate cross-linguistic research, including other Slavic languages. Students work in groups on specific projects involving recording and transcribing children’s speech in the CHILDES universal format and data collection tasks in the LABLASS system. The Database for Spoken German (DGD) is used in the German Linguistic department at the University of Mannheim to teach BA and MA-level students how to analyse the usage of interaction signs, such as interjections and compare their frequency to written language data, such as postings on Wikipedia talk pages that are available via the Corpus Search, Management and Analysis System (COSMAS II). The latter is often used for cross-lingual studies. Students also explore collocations and create word development curves and word profiles with the help of corpus tools. Teachers teaching Dutch as a second language should look at the OpenSoNaR corpus, which contains written and spoken corpora of Dutch from the Netherlands and Flanders (500 million words). The corpus is used for linguistic and human language technology research, and the development of NLP applications. It is easily accessible through a user-friendly interface that offers basic and advanced functionalities for corpus queries, including regular expressions. OpenSoNaR Search Interface Tutorials in Dutch are available in open access on Surf.nl, and video recordings on Vimeo. You can access the corpus using your university credentials; otherwise, apply for a CLARIN ERIC account. References:blank
• Computer-Mediated Corpora (CMC)
• L2 Learner Corpora
• Comparable Corpora
• Parallel Corpora
• Multilingual Web Corpora
• Spoken Corpora
If you want to learn more about speech technologies, we recommend the Automatic Speech Recognition and Forced Alignment learning block on Moodle, designed by Louis ten Bosch and Henk van den Heuvel from Radboud University, Nijmegen.
To sum up, the resource families provide an excellent opportunity for teachers and educators to enhance their students’ learning experience through “data-driven learning” (Perez-Paredes et al., 2019). Many of the corpora listed in the resource families are open and available via concordancers, which makes them an excellent open educational resource. This allows for a broader scope of instruction beyond conventional materials.
References:
- Bernardini, S. (2006). Corpora for translator education and translation practice: achievements and challenges. In Proceedings of LREC 2006 (5th Language Resources and Evaluation Conference), pp. 17 – 22.Paris: ELRA.
- Deshors, S.C. (2021). Corpora in applied linguistics. In H. Mohebbi & C. Coombe (Eds) Research Questions in Language Education and Applied Linguistics, pp. 805-809. Cham: Springer.
Paquot, M. & S.T. Gries (Eds). A Practical Handbook of Corpus Linguistics. Cham: Springer. - Pérez-Paredes, P. (2022). A systematic review of the uses and spread of corpora and data-driven learning in CALL research during 2011–2015. Computer-Assisted Language Learning 35: 36-61.
If you find resources in the VLO or the Resource Families that you would like to bookmark for later exploration and use, you could add the URL or persistent identifier to a virtual collection using the Virtual Collection Registry (VCR). Virtual collections can be shared with others and cited using persistent identifiers, e.g. handle and/or DOI. Furthermore, if the collection contains plain text files, you can process them directly with Language Resource Switchboard for annotation, translation, or visualisation. Last but not least, the metadata of the collections can be explored with the CMDI Explorer and downloaded in HTML or JSON format. See the quick guide below to learn how to create a virtual collection.
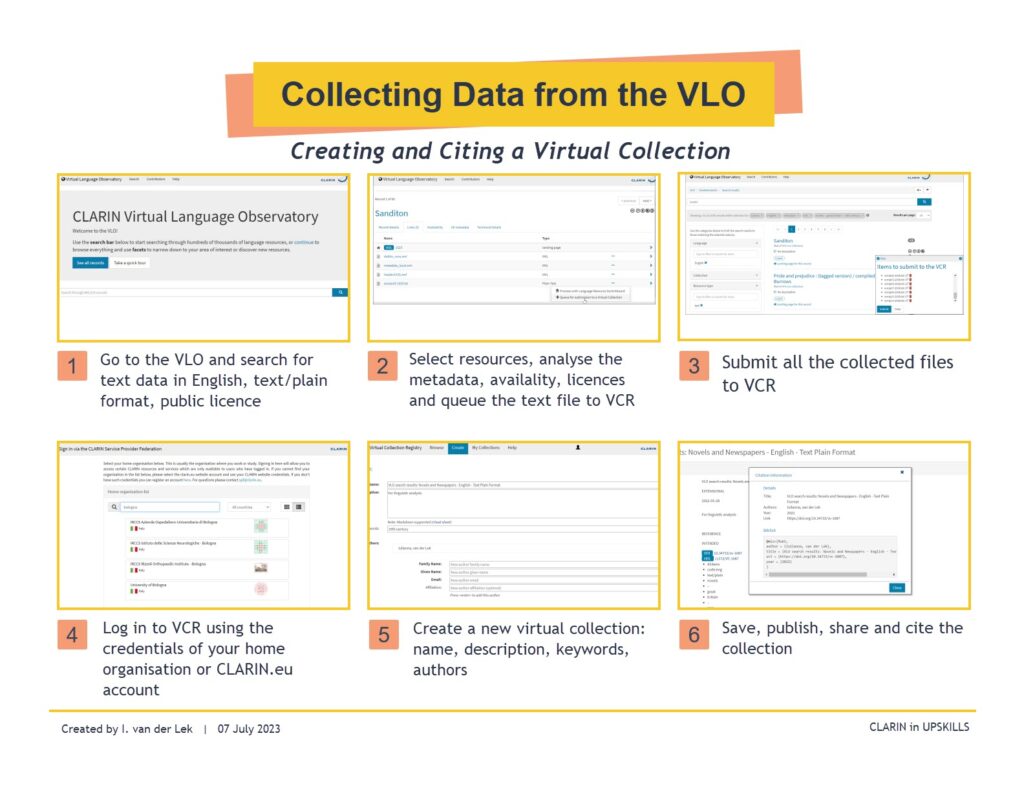
Collecting Data from the VLO and Creating a Virtual Collection
When used in the classroom, the VCR can be useful in the following scenarios:
-
- Before the class, the VCR can be used to create a collection around a specific topic that you would like to teach, e.g. links to exemplary datasets, tools and tutorials that you would like to share with the students. For example, here are a few virtual collections that have been compiled for demonstration purposes:
- A collection of books, articles, tutorials and guidelines on Research Data Management in linguistics: DOI 10.34733/vc-1078.
- A collection of tutorials and user guides for the NLP tools available via the Language Resource Switchboard: DOI 10.34733/vc-1079.
- A collection of Jane Austen’s works in plain text format, which can be used for linguistic analysis with Switchboard tools, or downloaded and used to compile a corpus with corpus building tools, such as BootCat or SketchEngine: DOI 10.34733/vc-1080.
- When students need to collect language data for a specific project, they could use the Virtual Language Observatory or other CLARIN national repositories to search for datasets, digital text collections, and corpora, add them to a virtual collection and save them for later analysis. They can then share the collection with the teachers, who can evaluate the metadata quality and give feedback on the selection of resources. The collections can then be cited with the help of persistent identifiers. Reusing digital texts from repositories and adding them to a virtual collection may save some time because the students do not have to manually search the Web, download, convert files to text format and note down each file’s metadata in a spreadsheet.
- Before the class, the VCR can be used to create a collection around a specific topic that you would like to teach, e.g. links to exemplary datasets, tools and tutorials that you would like to share with the students. For example, here are a few virtual collections that have been compiled for demonstration purposes:
📖 Teaching and Learning Resources on Moodle Introduction to Language Data: Standards and Repositories Use the following interactive presentation to teach students how to create and cite virtual collections:
|
Nowadays, there is a wide variety of applications to discover, explore, analyse and annotate language data, and it might not always be easy to select a tool suitable for teaching language data science. In CLARIN, you can find tools via the following paths:
-
- The Virtual Language Observatory is a discovery platform not only for language resources and digital text collections but also software and various tools, e.g. source code for open-source translation tools (e.g. LINDAT Translation service), language models for training machine translation engines (e.g.https://hdl.handle.net/11234/1-3732), corpus taggers, term extraction tools, OCR tools and file conversion tools.
- The Language Resource Switchboard connects to several NLP tools developed within the infrastructure, and it can be used to find a matching tool for a specific text type and language. More details about this service are provided in the section below.
- The CLARIN Resource Families contain both corpora and corpus query tools and a curated collection of NLP tools for normalisation, named entity recognition, PoS tagging , lemmatisation and sentiment analysis.
- Because not all tools developed in the CLARIN member countries are discoverable via the services listed above, we recommend you also check your national consortia’s websites.
Below, we briefly introduce Language Resource Switchboard and point to other useful NLP tools and applications that are often used in educational settings:
If you are new to incorporating natural language processing tools in your classroom and uncertain about which tools to choose for text or file processing and analysis, exploring the Language Resource Switchboard could prove beneficial. The service can process digital text collections found via the Virtual Language Observatory or other research data repositories (e.g. TextGrid) with matching NLP tools available through the infrastructure. Below, you will find the list of tools that connect to the Switchboard. Note that some tools require you to authenticate with your institutional credentials. NLP tools accessible via the Language Resource Switchboard Before using Switchboard, you may need to introduce students to basic NLP methods for text analysis. On Moodle, you will find a basic tutorial showing the main types of automatic annotation methods useful for linguists, e.g. text segmentation, tokenisation, part-of-speech tagging, syntax/parsing, named entity recognition, and sentiment analysis. Teaching and Learning Resources on Moodle: Introduction to Language Data: Standards and Repositories The quick guide below shows how you can process a plain text file from the VLO with Switchboard directly from the search results interface. In Switchboard, depending on the level of the students and the task you want to demonstrate, you can select UDPipe for automatic NLP tasks or choose Voyant Tools to introduce students to basic text analysis in a visually appealing way. If you need to teach more advanced corpus query functionalities, you can also export the file from the repository where it is located and upload it to AntConc or SketchEngine. Finally, you can cite the language resource with the handle assigned by the repository. Text processing and Analysis with Switchboard Below, we highlight some tools accessible directly from Switchboard that may be suitable for classroom use. Corpus Analysis and Visualisation We have already presented a few available corpora for browsing and linguistic exploration in tools such as NoSketch Engine, Korp, and KonText. We would now also like to mention Voyant Tools, which are directly accessible via the Language Resource Switchboard. Voyant Tools is a user-friendly web-based reading and analysis environment for digital texts offered by CLARIN-DK and is suitable for teachers and students with no technical background. The tools can also be downloaded and run on local computers. Teachers and students can use this online environment to analyse automatic text with functionalities such as word frequency lists, frequency distribution plots and KWIC displays. Students can use the tools to analyse an online collection of journals, blogs, and websites and include the link to their analysis directly in their research reports (if these are published online) so that the readers can view the results. 👉 PRO-TIP: See the Resource Families of Corpus Query Tools if you are looking for more corpus-query tools and platforms. The overview includes desktop and online applications, covered languages, and links to user guides. Automatic Annotation of Texts UDPipe is a language-independent software that produces custom annotation pipelines for tokenisation, tagging, lemmatisation and dependency parsing of CoNLL-U files. The tool is based on the Universal Dependencies (UD) framework, which produced about 200 treebanks (consistently annotated by a human) for over 100 languages (Zeman et al, 2023). The framework is very popular because it is intuitive and does not adhere to any formal theory. LINDAT CLARIAH-CZ infrastructure provides UDPipe as a free web service for testing services. UD and the CoNLL-U format are also supported by other annotation tools, such as the BRAT rapid annotation tool, WebAnno, and SketchEngine. An international team of volunteers maintains the framework, and everyone can join them to start building their own corpus through the TEITOK platform. UDPipe and the TEITOK UD 2.11. corpus are used in the NLP courses at the Institute of Formal and Applied Linguistics at Charles University for teaching MA students morphological analysis. Students acquire NLP skills by participating in real-life research projects related to the development and training of machine translation engines, corpora, lexicons, speech and dialogue systems, and text processing and semantics. WebLicht (“Web-based Linguistic Chaining Tool”; hosted by the CLARIN centre at the University of Tübingen) is a user-friendly web service that can be used to teach and demonstrate automatic annotation of texts in English, German, Dutch, French, and Italian. It is included in the practical lab sessions on Corpus Linguistics at the University of Saarland, Germany, to teach corpus annotation. The service is integrated with the CLARIN infrastructure and accessible from Switchboard, Federated Content Search and the Virtual Collection Registry. Several NLP tools (e.g. sentence splitting, tokenisation, lemmatisation, POS tagging, morphological analysis, named entity recognition, dependency parsing, constituency parsing) are integrated to help researchers create and visualise custom processing chains easily. See below for an example of dependency parsing in WebLicht. The workflow is explained in detail in Hinrichs, M. (2022). Users can access the service through their academic institutions. Dependency parsing in WebLicht Teaching and Learning Resources on Moodle: Introduction to Language Data: Standards and Repositories Use this tutorial from Moodle to teach students how to annotate a raw collection of text corpora from the GitHub repository with WebLicht. 👉 PRO-TIP: If you are searching for ready-made training materials to introduce your students to UD, check the following learning resources: Manual Annotation of Texts WebAnno is a user-friendly open-source web-based annotation tool for manual linguistic annotation tasks (e.g. morphological, syntactical, and semantic annotations) that can be introduced in teaching at the BA and MA level to introduce the students to annotation. The teacher can conduct multiple annotation projects in parallel and assign students different roles, e.g. annotator, curator, or project manager. Students can annotate in groups following the full annotation workflow, as in the figure below. Machine learning capabilities are integrated to make the work of the curator/reviewer easier. For example, WebAnno learns from pre-annotated data and makes suggestions. The annotator can accept or reject the suggestions with a single click. The suggestions help improve the training data. The project manager can assign workload to annotators and monitor their projects. The tool is also offered as a service to members of research institutions, e.g. CLARIN-D and Fin-CLARIN. Workflow of a WebAnno project (Castilho, 2014) INCEpTION is an upgraded version of WebAnno that is supported by UKP Lab at TU Darmstadt. This text-annotation platform offers a user-friendly interface for various collaborative semantic annotation tasks on written text, mostly for linguistic and machine-learning purposes. The platform is available both in a desktop version and online, and it includes a recommender system to assist in creating annotations quickly and easily. Additionally, it enables corpus creation by searching external document repositories and importing documents. Due to its user-friendly interface, user guide and tutorials, it can be used in the classroom to create semantic annotation projects with the students. INCEpTION is available as a service to CLARIN-EL members. Examples of annotations in INCEpTION Annotation of Speech WebMaus is a web service developed by the Bavarian Archive for Speech Signals, and it can be used to automatically align transcriptions and speech signals. The results can be used in Praat. The service is part of the suite of BAS web tools for speech processing and provides word and phoneme alignment for more than 25 languages. Watch the short video tutorial that follows: https://www.youtube.com/watch?v=7lI-gOShtFA Teaching and Learning Resources on Moodle To learn more about automatic speech recognition, see the UPSKILLS Learning content on Moodle: After getting acquainted with some of the tools in the Switchboard, access the service and try it. You can do so by following the steps in the quick guide that follows. Remember that the resources in plain text format from the Virtual Language Observatory can be submitted to Switchboard directly from the VLO interface. Finding NLP Tools in Language Resource Switchboard Note also that: Finally, Switchboard can also be accessed from other research data repositories, e.g. CLARIN VLO, CLARIN Virtual Collection Registry, ARCHE, DARIAH-DE Repository, PARTHENOS VRE, and TextGrid. Teaching and Learning Resources on Moodle Introduction to Language Data: Standards and Repositories: Use the following presentations and tutorials to teach students how to use the Switchboard tools to process digital text collections: Presentations: Tutorials: If you are interested in teaching students how to compile and analyse large text corpora using programming languages but you do not know where to start, check these UPSKILLS courses on Moodle: Jupyter Notebooks provide a popular environment for programming, especially in educational contexts, to teach coding and concepts such as topic modelling. To understand how they can be used both for research and teaching, we recommend Quinn et al. (2019). The tutorial demonstrates how to write a Jupyter notebook for data analysis as part of a research project and then adapt it for classroom use. The CLARIN centres have also implemented notebooks in their infrastructure to support researchers in data analysis as part of their research projects. You can find a collection of notebooks here. For example, Portulan CLARIN has implemented various NLP tools via a workbench (e.g. quantitative tools for syntax) and includes examples and documentation to help researchers and students design an experiment with Jupyter Notebooks (A great collection of open-source course materials on various NLP topics, including the use of Jupyter notebooks in educational settings, is available on GitHub). CLARIN Center of Estonian Language Resources has developed the EstNLTK python package for processing Estonian, as well as a series of tutorials in Jupyter Notebooks on different NLP components, such as text segmentation, morphological processing, syntactic analysis, word embeddings, etc. For educational materials in Estonian, please check the NLP course taught at the University of Tartu, available on GitHub. EstNLTK can be installed in Google Colab, which implements Jupyter Notebooks within the Google suite. This allows you to create a document containing executable code, which is stored on your GDrive and can be shared with peers for editing and commenting. It may be a more user-friendly option than GitHub for classroom use. If you want to learn more about EstNLTK, see this post in Tour de CLARIN. Other helpful teaching and learning resources are: 👉 PRO-TIP: After acquiring basic programming skills, learn how to use Jupyter notebooks and CLARIN NLP tools to process large collections of texts from Virtual Language Observatory with the help of this tutorial: References: All the language technologies introduced in this section can support teaching and research across various disciplines to teach students how to develop a data-driven mindset and draw insights and conclusions from large volumes of text. While evaluating various NLP tools for classroom use, it is beneficial to collect feedback from students on the usability of the tools used and the infrastructure in general to inform both the tool developers and other teachers who may be interested in using the same tools in the classroom. The following criteria could be used to evaluate your experience with the tools and collect insights from students. All the feedback, suggestions for improvements or additional features could then be shared with the infrastructure and tools developers to improve the subsequent versions of their tools. blank
• Using the Language Resource Switchboard for Text Processing
• Other NLP Tools and Applications
• Collecting Feedback
This section is relevant for teachers who plan to include essential FAIR-research data management skills in their linguistic, translation and other language-related courses or programmes. As demonstrated throughout this guide, if language resources and tools are documented well, curated, published and archived in a discipline-specific research data repository, they are easy to find, validate and reuse for research and educational purposes. Moreover, research data management and responsible data sharing have become mandatory in doctoral programmes and for researchers participating in Horizon Europe projects. Therefore, various initiatives at the EU level, such as EOSC and FAIRsFAIR recommend that universities include basic skills for open science and research data management across all domains, disciplines and levels. The integration of research data management is generally achieved by following the FAIR guiding principles for data management, which ensures that research data is findable, accessible, interoperable, and reusable. Nevertheless, there are still numerous challenges due to the lack of consensus around the notion of “data’’, especially in linguistics (Good, 2022).
Traditionally, arts and humanities programs have yet to emphasise the importance of research data management and computer literacy skills. However, in contemporary education, marked by increasing interdisciplinarity, such skills are becoming indispensable regardless of one’s career path. As many lecturers have already pointed out during UPSKILLS multiplier events and testimonials, students of language and linguistics often struggle with basic computer skills at the MA level, e.g. organising data in an Excel file, recognising the difference between basic file formats, zipping and unzipping files, and/or understanding technical concepts, such as “annotation’’. This lack of basic computer literacy at any level of study can impact the students’ learning experience and the lecturer’s initial course design and plan (Simonovic et al., 2023a). Moreover, if one does not have basic computer skills, one will encounter challenges when managing their research data, e.g. downloading language resources from repositories in various formats, unzipping them, uploading them to another tool, or collecting language data from human participants, organise, transcribe, annotate, store it in a safe place.
To prepare language and linguistics students to work with research data and engage in data-driven projects, they can be recommended to take an introductory ICT course at the start of their BA programme and a general research data management course after they learn what research data is. However, training activities addressing RDM and FAIR data skills are still lacking at the bachelor and master levels (Stoy et al., 2020). Usually, RDM courses are offered via the university library, are not discipline-specific and often target students in doctoral programmes.
👉 PRO-TIP: If your institute does not offer general RDM courses, curriculum designers refer to the case studies documented in Good Practices in FAIR Competence Education (Garbuglia et al., 2021) for inspiration on designing such programmes. Additionally, please check Existing Learning Materials Surveyed Within UPSKILLS and the SSH Training Discovery Toolkit for available training resources in open access. |
Moreover, in the first years of their BA studies, students can be introduced to basic data management skills by simply teaching them how to organise their files on their computers in a meaningful way, handle their data carefully, share files responsibly via secure cloud-based platforms, perform regular backups and archive their work at the end of a project or semester for possible future reuse.
After students have acquired basic data management skills, teachers can engage them in domain-specific (research-based) data-driven projects, e.g. produce a dataset to answer a research question, add new annotation types to an existing corpus, compile a corpus for an under-resourced language, or develop a language model. Students can work on such projects collaboratively in the classroom, remotely, or as part of their final BA/MA theses under the teacher’s supervision. The CLARIN infrastructure can be used to find guidance on standards, formats, and how to handle legal and ethical issues when the projects involve working with personal and sensitive data. At the end of the project, teach students how to find a suitable repository for their data and deposit, publish and share their corpus with the research community.
For guidelines on depositing general research outputs and language resources in a research data repository, see Section 6 in the Guidelines for Student Projects and Research Reporting Formats (Simonovic, M. et al., 2023b).
It often takes students time and effort to understand the current interpretations of the legal requirements and to try and fulfil all of them, and they need individual help with this. International deposits can be problematic (if copyrighted or sensitive data is collected from outside the EU and deposited within the EU or when using (possibly sensitive) data from old research projects). If the data can be public and open and the licenses have been cleared, the student can deposit the data independently, and all is well. (Anonymous lecturer, UPSKILLS Questionnaire of Lecturers)
To teach students how to create a research data management plan in language and linguistics and deposit, share and archive their language resources, see the following learning and teaching resources on Moodle:
📖 Teaching and Learning Resources on Moodle Introduction to Language Data: Standards and Repositories UNIT 1: Introduction to the Language Resource Lifecycle and Management
Unit 5: Legal and Ethical Issues in Language Data Collection, Sharing and Archiving
Unit 6: Student Project
|
👉 PRO-TIP: For other examples of how RDM practices have been integrated in linguistics research, we recommend the chapters and case studies from The Open Handbook of Linguistic Data Management (Berez-Kroeker et al. 2022). The handbook shares best practices for managing, archiving, sharing, and citing linguistic research data. Although the case studies may be too advanced for the BA level, they could be discussed at the MA and PhD levels. The online companion course has interactive quizzes and learning activities, which you can use and adapt freely for non-commercial educational purposes. |
References:
- Berez-Kroeker, A. L., H.N. Andreassen, L. Gawne, G. Holton, S.S. Kung, P. Pulsifer & L.B. Collister – The Data Citation and Attribution in Linguistics Group, & the Linguistics Data Interest Group. (2018). The Austin Principles of Data Citation in Linguistics (Version 1.0). https://site.uit.no/linguisticsdatacitation/austinprinciples/
- Garbuglia, F., B. Saenen, V. Gaillard & C. Engelhardt. (2021). Good Practices in FAIR Competence Education (1.2). Zenodo. DOI: 10.5281/zenodo.6657165
- Good, J. (2022). The scope of linguistic data. In A.L. Berez-Kroeker, B. McDonnell, E. Koller & L.B. Collister (Eds.) The Open Handbook of Linguistic Data Management, pp. 27-48. Cambridge, MA: MIT Press Open.
- Kung, S.S. (2022). Developing a data management plan. In A.L. Berez-Kroeker, B. McDonnell, E. Koller & L.B. Collister (Eds.) The Open Handbook of Linguistic Data Management, pp. 101-115. Cambridge: MIT Press Open.
- Simonović, M, I. van der Lek, D. Fišer & B. Arsenijević, B. (2021). Guidelines for the students’ projects and research reporting formats. Zenodo. DOI: 10.5281/zenodo.8297430
- Simonović, M., B. Arsenijević, I. van der Lek, S. Assimakopoulos, L. ten Bosch, D. Fišer, T. Kraš, P. Marty, M. Miličević Petrović, S. Milosavljević, M. Tanti, L. van der Plas, M. Pallottino, G. Puskas & T. Samardžić. (2023). Research-based teaching: Guidelines and best practices. Zenodo. DOI: 10.5281/zenodo.8176220
- Stoy, L., B. Saenen, J. Davidson, C. Engelhardt & V. Gaillard. (2020). FAIR in European Higher Education (1.0). Zenodo. DOI: 10.5281/zenodo.5361815
To target basic RDM skills at BA, MA and PhD levels, we recommend following the general guidelines in FAIRsFAIR Teaching and Training Handbook for Higher Education Institutions (Garbuglia et al. 2021). This handbook provides a map of FAIR skills and competences for all levels of study, along with practical implementation guidelines, lesson plans, and learning outcomes. Please note that the learning outcomes are domain-independent and must be adapted to a specific discipline.
According to the authors, students at the Bachelor level (the level targeted in UPSKILLS) should acquire the following basic competetences when working with research data:
-
- Can paraphrase the concepts of Open Research, Open Access, and Open Data and explain their benefits
- Can paraphrase the FAIR principles in data management and recognise the relationship between FAIR, Open and RDM
- Can define what Research Data Management is and explain its benefits
- Can develop a basic data management plan for their work, and identify different types of data documentation
- Can identify, describe and use different types of metadata, formats and standards
- Can identify, describe and use metadata registries to find data (e.g. Virtual Language Observatory)
- Can explain what a trusted data repository is and how to find it; can compare different certifications for data repositories (e.g. CoreTrustSeal vs CLARIN certification)
- Can explain the importance of data discovery and reuse
- Can recognise, explain and use persistent identifiers to access data and other resources
- Can identify and use different levels of data security, protection and backup
- Can explain basic rules and regulations for handling personal and sensitive data and know how to comply with them
- Can summarise and explain ethical principles and responsible data use (e.g. CARE principles) and identify potential legal issues around data use, management and sharing
- Can explain the research data lifecycle and compare different models
- Can explain the role of ontologies and vocabularies in data discovery, identify and use domain-specific ones
To include RDM learning outcomes in a domain-specific course or programme, pick the ones from the list that match the overall course goals and objectives, and adapt them to the type of research project and language data the students will work with during the course. As examples, see how RDM practices have been integrated into the learning outcomes of the UPSKILLS learning content and guides:
- Unit 1, 2, 4 and 6 of the learning block, Introduction to Language Data: Standards and Repsositories on Moodle
- Part A, 6 of the Research-Based Teaching: Guidelines and Best Practices (Simonovic, M. et al, 2022)
- Section 2 of the Guidelines for Student Projects and Research Reporting Formats (Simonovic, M. et al., 2021)
References:
- Garbuglia, F., Saenen, Bregt, Gaillard, Vinciane, & Engelhardt, Claudia. (2021). D7.5 Good Practices in FAIR Competence Education (1.2). Zenodo. https://doi.org/10.5281/zenodo.6657165
- Simonović, M, I. van der Lek, D. Fišer & B. Arsenijević, B. (2021). Guidelines for the students’ projects and research reporting formats. Zenodo. DOI: 10.5281/zenodo.8297430
- Simonović, M., B. Arsenijević, I. van der Lek, S. Assimakopoulos, L. ten Bosch, D. Fišer, T. Kraš, P. Marty, M. Miličević Petrović, S. Milosavljević, M. Tanti, L. van der Plas, M. Pallottino, G. Puskas & T. Samardžić. (2023). Research-based teaching: Guidelines and best practices. Zenodo. DOI: 10.5281/zenodo.8176220
For exemplification, we include the outline of the CLARIN student project designed in collaboration with the University of Bologna for the UPSKILLS learning content block: Introduction to Language Data Standards and Repositories. The full description of the project, instructions for students, templates and guidelines are available on Moodle, Unit 6. Student Project.
Title: Designing, Compiling and Archiving a Corpus of Bank Bulletins Research Question: How do linguistic and communicative strategies differ between original and translated economic bulletins within the banking and financial sectors across various EU member states, considering institutional factors such as language diversity, cultural influences, and publication dates? Imagine you have been commissioned to collect a corpus of quarterly economic bulletins in the framework of an EU-wide effort to analyse and compare institutional communication strategies in the economic, financial, and banking domains. You are part of an international team of linguists who will build corpora from as many national central banks as possible, and the corresponding texts by the European Central Bank. You can either construct a corpus of ECB bulletins (in English or another of the EU official languages) or national bank bulletins for a country of your choice (in English or in the country’s official language). The following metadata is considered relevant by your commissioners:
You are encouraged to provide further metadata that you consider relevant. The corpus should contain at least 50 texts in plain text format. Linguistic annotation is an optional task for extra points. The commissioner requires that the corpus is deposited in a plain-text format in a domain-specific certified data repository and that it can be shared with the other linguists via a Creative Commons Licence. Workload: 1-2 ECTS (depending on the tasks that the teacher will choose to include) Level: BA Learning Outcomes: By the end of this project, the students will be able to:
Prerequisites: To be able to work on this project independently, students first follow the following learning blocks on Moodle:
Recommended Background Knowledge
Reporting Format
Assessment The project could be evaluated in the following way:
Templates and Guidelines
Designers: Iulianna van der Lek (CLARIN ERIC) and Silvia Bernardini (UNIBO) Reviewers: Novella (UNIBO), Darja Fišer (CLARIN ERIC), Marko Simonovic (UGraz) and Francesca Frontini (CLARIN ERIC) |
For more examples, see the 16 research-based courses we piloted in UPSKILLS, which include specific learning outcomes related to using research infrastructures, repositories, corpora and tools.
🏗 Research Tracking Tool
As part of the infrastructures deliverable, our partners from the University of Zurich have developed an interactive research tracking tool that enables students to track their progress during projects. At the same time, teachers can provide feedback at intermediate stages throughout the project.
At each stage of their project, students should fill in the template using the student version of the tool. Teachers can then use the teacher’s version of the tool to provide brief feedback in the designated field for each version submitted by the students. The research tracker’s versions can be downloaded below:
💾 UPSKILLS Research Tracking Tool (Student edition)
💾 UPSKILLS Research Tracking Tool (Teacher edition)
🖇 List of Open Corpora (Petnica edition)
We compiled a list of open corpora for the student projects taught at UPSKILLS Summer School in Petnica, Serbia, in July 2023, which may also be useful for other teachers and students. Except for Armenian, Bengali, and Chinese corpora, all other corpora can be directly accessed and queried via NoSketch Engine (clarin.si), which is the open-source variant of the commercial SketchEngine corpus query tool, and implemented by the CLARIN Slovenian repository for language resources. If you prefer to use the corpora in another concordance tool, you can download them from the CLARIN.SI repository.
- Armenian and Bengali: W2C (Web to Corpus) Corpora (available in the LINDAT/CLARIAH-CZ repository; they can be queried in the KonText concordancer)
- Catalan: SpanishParlaMint-ES-CT 3.0
- Chinese: Sheffield Corpus of Chinese, The Lancaster Corpus of Mandarin Chinese (available in the Oxford Text Archive Repository)
- Croatian: CLASSLA-web.hr, CLASSLAWiki-hr, ENGRI, ParlaMint-HR 3.0
- English: ParlaMint-GB 3.0, EU DGT-UD (English), ukWaC
- French: frWaC LeMonde: francosko, ParlaMint-FR 3.0
- German: deWaC, EU DGT-UD (German), ParlaMint-AT 3.0
- Italian: EU DGT-UD (Italian), itWaC, ParlaMint-IT 3.0
- Macedonian: CLASSLAWiki-mk
- Polish: EU DGT-UD (Polish), ParlaMint-PL 3.0
- Serbian: CLASSLA-web.sr, CLASSLAWiki-sr, ParlaMint-RS 3.0, SETimes.SRReLDI-sr, CLASSLAWiki-sh
- Slovenian: CLASSLA-web.sl, CLASSLAWiki-sl, EU DGT-UD (Slovenian)
🫂 Get Involved in the Community
Teachers and educators unfamiliar with CLARIN can engage with the infrastructure in the following ways:
- Join the CLARIN Trainers’ Mailing List and collaborate with the Trainers’ Network to exchange and discuss training and educational initiatives involving language data science and find new opportunities for collaboration.
- Adapt, Translate, Create and Share Training Materials: Teachers and trainers who use this guide and adapt any of the UPSKILLS learning content for the classroom are invited to share their experience via this CLARIN training and education call, part of the CLARIN Annual Conference. Each year, a session showcases new educational initiatives and training materials on different language research topics.
- Contribute to the Digital Humanities Course Registry: Teachers and trainers in Digital Humanities are encouraged to register the metadata of their courses and training programmes in the Digital Humanities Course Registry. The DH Course Registry is a joint effort of the CLARIN ERIC and DARIAH-EU research infrastructures. It helps students, researchers, lecturers, and institutions to discover, promote, and connect to DH teaching and training activities.
- Engage in Outreach Programs and Events: Participate in the CLARIN Cafés where lecturers and students meet informally to exchange and discuss specific topics related to language research. Additionally, the Impact Stories can be used in the classroom to show how language resources and technologies are used to tackle societal issues.
- Encourage Student Involvement: IInvolve students in community engagement activities, such as participating in online Cafés, hackathons and summer schools, and presenting their projects and ideas at the CLARIN Annual Conference. The conference includes a session for PhD students who use the CLARIN infrastructure in their research and would like to receive feedback on their work.
We welcome feedback from the users of these guidelines. Also, teachers and trainers using corpora and NLP tools from the CLARIN infrastructure (and not only) are invited to contribute their examples of teaching and learning activities to us.